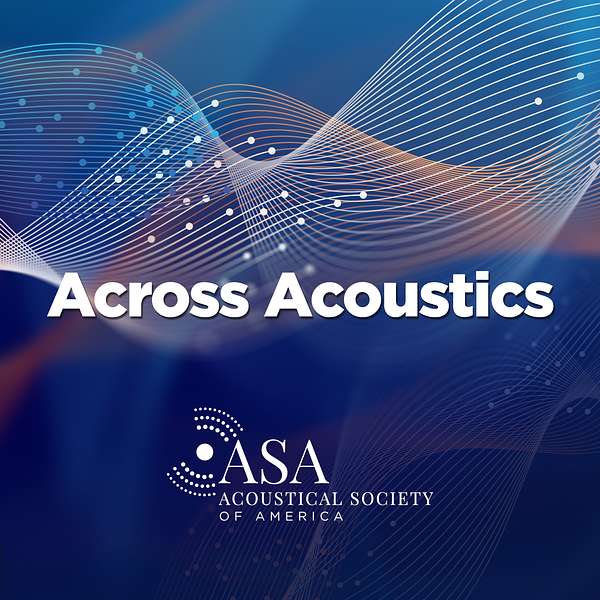
Across Acoustics
Across Acoustics
Why don't speech recognition systems understand African American English?
Most people have encountered speech recognition software in their day-to-day lives, whether through personal digital assistants, auto transcription, or other such modern marvels. As the technology advances, though, it still fails to understand speakers of African American English (AAE). In this episode, we talk to Michelle Cohn (Google Research and University of California Davis) and Zion Mengesha (Google Research and Stanford University) about their research into why these problems with speech recognition software seem to persist and what can be done to make sure more voices are understood by the technology.
Associated paper: Michelle Cohn, Zion Mengesha, Michal Lahav, and Courtney Heldreth. "African American English speakers’ pitch variation and rate adjustments for imagined technological and human addressees." JASA Express Letters 4, 047601 (2024). https://doi.org/10.1121/10.0025484.
Read more from JASA Express Letters.
Learn more about Acoustical Society of America Publications
Music: Min 2019 by minwbu from Pixabay.
Kat Setzer 00:06
Welcome to Across Acoustics, the official podcast of the Acoustical Society of America's publications office. On this podcast, we will highlight research from our four publications. I'm your host, Kat Setzer, editorial associate for the ASA.
Kat Setzer 00:19
I imagine most of you had had the joy of interacting with a virtual assistant like Siri or Alexa. Today we're going to talk a bit about the speech recognition systems used in these assistants and other technology. Here with me are Michelle Cohn and Zion Mengesha, whose article, "African American English speakers’ pitch variation and rate adjustments for imagined technological and human addressees," recently published in JASA Express Letters and was featured in an AIP Publishing Scilight. Thanks for taking the time to speak with me. How are you?
Michelle Cohn 00:52
Great, yeah. Thanks so much for having us.
Zion Mengesha 00:54
Doing great. So happy to be here with you today.
Kat Setzer 00:57
Fantastic. So first, just tell us a bit about your research backgrounds.
Michelle Cohn 01:01
Yeah, so I'll start. My name is Michelle Cohn. I'm a postdoc researcher in the UC Davis phonetics lab, and for the last two years, I was also a visiting researcher with Google Responsible AIUX. And my research focuses on how people talk to, perceive, and learn from voice technology, like Siri and Alexa. And I'm a linguist by training, so one of the core values in linguistics is really an appreciation for linguistic diversity in accents, dialects, varieties and languages all over the world.
Zion Mengesha 01:27
Hi, I'm Zion Mengesha, and I'm in my final year of my PhD at Stanford in linguistics. I'm a sociolinguist, so a lot of my interest is in sociolinguistics and in production-- in particular, the connection between language, gender, race and ideology. So I've done fieldwork in Sacramento, looking at how social and political ideology shape how African American women speak. And then at Stanford I've also been a part of the Voices of California project, which is a dialectology project, looking at how different people in different areas of mostly the Central Valley of California speak. And this is a project led by Dr. Penny Eckert and Dr. Rob Podseva. And then a lot of my work has also been about bringing a sociolinguistic perspective to technology, and understanding the costs and consequences of dialect discrimination towards African American English in speech recognition and large language models. So with Michelle, I also worked for three and a half years on Google's responsible AI and human centered technology team, collaborating with social psychologists, computer scientists, and engineers to find ways to make these systems more fair.
Michelle Cohn 02:37
Finally, we just want to give a shout out to our two other amazing co authors, Michal Lahav and Courtney Heldreth, who are Google researchers focused on access and equity.
Zion Mengesha 02:48
Yes, and we couldn't have done this work without them.
Kat Setzer 02:51
That is so awesome. Yeah, it sounds like this is such an important research right now. And so what we're talking about today is exactly what Zion was just referencing, having to do with voice technology like automatic speaker recognition systems that convert speech to text. Can you explain how these systems typically work?
Michelle Cohn 03:07
Sure. So speech recognition consists of training data sets of speakers, so mapping the acoustic patterns for a given word, like the word "cat," onto the word. So there's really a tremendous amount of variation in spoken language. So each time you produce the word cat, it's slightly different. So you could say, "cat," you could say, "cat," you could say "cat." And it varies even within a single person. And then beyond that, there's also variation by age, gender, region, race, and ethnicity. And so while these systems are getting better, they do make mistakes during this mapping, especially for language varieties they're not familiar with. And so this really parallels the types of misunderstandings a person might have if they hear a new accent or dialect, but they don't have that exposure or you could think of it as training data.
Kat Setzer 03:50
Oh, yeah, that makes a lot of sense. So, in addition to that, research typically shows that folks change their speech when talking to these systems. Why does that happen?
Michelle Cohn 03:59
Yeah, I've been exploring this in my postdoc research and a bit at Google. And we've really been finding that people adjust their speech in distinct ways when they talk to voice technology versus humans. So people are often louder and slower when talking to Siri and Alexa and Google Assistant than when they talk to a human voice. And these are similar types of adjustments that we see for other types of listeners, but also in background noise. And so it seems to suggest that speakers perceive that there's a barrier in their communication, and they're adapting to overcome that barrier.
Zion Mengesha 04:32
Yeah, and I'll also add in some of our work at Google, and specifically, I'm referencing a paper we published in Frontiers in Artificial Intelligence, we found that a majority of African Americans believe that the technology doesn't understand the way that they speak and that the technology was not made for them. So one of our participants in that study from Chicago said, "The technology is made for the standard middle-aged, white American, which I am not." Most of our participants, and that's 93% specifically, reported that they modify their dialect in particular in order to be comprehended by voice technology.
Kat Setzer 05:07
Interesting, interesting. So what sort of changes do folks typically make in their speech when talking to Alexa, Siri, or another one of their AI pals?
Michelle Cohn 05:16
They're often louder, slower, sometimes we see differences in pitch-- so more or less pitch variation-- and a couple of studies, including this recent paper, we've been finding less pitch variation, which we think might be the speakers mimicking the perceived monotone text-to-speech voice, and that sounds, like, emotionless, if you think of Siri or Alexa. And so those are some of the features that we're tending to see. But really, that past research has really focused on mainstream varieties of English, so like California English, and really not looking at these varieties, like African American English, where our speakers are misunderstood at a much higher rate.
Kat Setzer 05:52
Okay. Okay. So what was the aim of this study?
Michelle Cohn 05:55
Yeah, so Zion, if you want to talk a little bit about your 2020 paper, it really, really changed the field and spurred up quite a bit of research in this area.
Zion Mengesha 06:04
Yeah. So in that paper, we explored differences and how the top five providers of speech recognition technology understood the speech of African Americans. And that data set comes from the corpus of regional African American language or CORAAL as well as white speakers in the voices of California project that I referenced earlier. And we found that there were significant differences, up to two times the word error rate. And so we find that speech-based technology misunderstood African American speech more. There were also some more nuanced findings. But I'll leave us there because that really set up our goals for this study, which was to understand what kinds of effects that might be having in how African Americans orient linguistically towards these systems.
Kat Setzer 06:55
Yeah, that's really interesting... and problematic, it seems like.
Zion Mengesha 06:58
Yeah, we've also been exploring some other work, how the experience of being misunderstood has emotional, behavioral, and psychological effects on African Americans. So it's certainly an important issue.
Kat Setzer
Right, right
Michelle Cohn 07:12
So the aim of this study was to look at how African American English speakers adapt their speech. And we looked at two features, so rate and pitch variation in technology- versus human-directed speech registers, and specifically wanted to see if the differences between technology- and human-directed speech are larger if the participant reports being misunderstood by ASR more frequently in their day-to-day lives.
Kat Setzer 07:40
Oh, interesting. Okay, so tell us about the setup of the study.
Michelle Cohn 07:45
Yeah. So together, we designed an experiment to test how African American English speakers adapt their speech in three imagined conditions: So imagining talking to a voice assistant, imagine talking to a friend or family member, or imagine talking to a stranger. And our goal was really to see if the adjustments speakers make for technology differed across these contexts. And then again, if they were misunderstood more commonly in their everyday life. In each of the three conditions, participants produced queries for the three types of addresses. So for example, they asked all three, how to get the weather for a future date in a specific location, or, for example, to call a friend. So they did the same types of queries for all three. And then we took measurements at the utterance level for each of these queries. So in total, they produced 51 productions toward each imagined addressee and then we compared these in our models.
Kat Setzer 08:35
Okay. Okay. What sort of errors did the study participants report having when interacting with speech technology previously?
Michelle Cohn 08:41
Yeah, so we were unfortunately not so surprised that participants actually had pretty similar experiences being misunderstood by technology. So the majority of participants reported being misunderstood by technology either most of the time or all of the time. So we didn't have a lot of variation in how often the ASR errors were occurring. But these ASR errors were specifically about the technology misunderstanding that African American English, so one participant said they have to retype their messages when they're texting. Another source of error is in family and friends' names, so it might call the wrong person. And so this can be really difficult. And two participants explicitly called out African American English as the reason why the technology isn't understanding them as they want.
Kat Setzer 09:27
Yeah, I can imagine that is incredibly, incredibly frustrating. So why did you focus on pitch variation and speech rate for the study?
Michelle Cohn 09:35
Yeah, so this is the first set of studies that we're doing on this data set, and these are two features that we've previously explored, and have come out pretty consistently in technology-directed speech adaptations. So for example, speakers are slower and more monotone when talking to an Apple Siri voice, but this really seems to vary across experiment paradigms, and also how expressive the human or TTS voice is, and so in this study, we really wanted to address that and have really an imagined context. We also wanted to control for real ASR errors that, as these participants showed, happen all the time for them. And so we wanted to control that and make sure those errors weren't happening in the interaction, so that way we could keep the three conditions parallel. And so we found, as we have in other work, we found that African American English speakers in the current study were also slower and produce less pitch variation when they were talking to an imagined voice assistant compared to talking to a friend, a family member, or a stranger. And so we found this was really exciting. Again, this was in the absence of any actual addressee. They're just sitting at home, imagining talking to a friend, they're imagining talking to a stranger or talking to a voice assistant, we see these really dramatic differences. And so that's exciting because we're starting to see kind of clusters of features that are popping up across technology- and human-directed speech studies. And we also looked at whether it, really determining the research question, whether how often they're misunderstood by technology, by ASR, mediates this relationship, but we found that it was really consistent-- but again, with the caveat that our participants are misunderstood by technology really frequently. So they have a more similar experience there. So it's possible with a wider participant group, we might see more variation or looking at speakers from different dialects, for example.
Kat Setzer 11:20
So you touched on this already a little bit, but how did the study participants adjust their speech when talking to the ASR systems when compared to talking to other humans?
Michelle Cohn 11:29
Yeah, so when they're imagining talking to a voice assistant, so voice assistants use automatic speech recognition, or ASR system, to transcribe the speech into written text and then process that. We found that when they're just imagining that, so they're imagine imagine talking to Google Assistant, or imagine talking to Siri or Alexa, their speech was slower. So that same utterance when they're asking to get weather in LA, on Thursday, right, that whole utterance, they say, "Assistant, get weather in LA on Thursday" was overall slower than when they ask a friend or family member a parallel question, "Hey, Dad, what's the weather gonna be like in LA on Tuesday?" or for a stranger, and then they also produce less pitch variation. So pitch variation is the changes, you can think about it as notes, so you can go higher, you can go lower. And basically, they were more monotone. So more like this, just flatter, when they were imagining talking to a voice assistant, or an ASR system, which we think could reflect their internalized representation of how those text-to-speech voices sound. So we have some other research showing that folks tend to rate text-to-speech voices as sounding not human, like emotionless, robotic and monotone. So it's consistent with that. We'll need to do more research to see what the sources are for that, but that appears to be kind of the cluster of effects that we're seeing in the current study.
Kat Setzer 12:54
So why do you suppose speakers of African American English have a harder time being understood by ASR systems? How do we get technology to adapt better to diversity in dialect?
Zion Mengesha 13:03
Those are such great questions. One of the largest limitations here is the data that ASR systems are trained on. And so I think that also leads into the answer to your second question, which is collecting more training data from African Americans that represent the heterogeneity or diversity in African American speech across the country. So one of the very exciting projects that's coming out of Google in a collaboration with Howard University between Dr. Gloria Washington and Dr. Courtney Heldreth, is project Elevate Black Voices are project EBV, in which they are indeed going across the country and collecting audio data from African Americans in order to build out what will be a publicly available dataset that ASR systems, including Google and others, can use to incorporate into their training models in order to improve understanding of African Americans. And I think that's the first step. We've heard also from African American users of technology that they want to see, or rather hear, speech technology that sounds more like them. And so also creating more ASR voices that are representative of African American English will also facilitate better communication between African American speakers and the technology, which is a part of what we are finding in already the differences between how African American speak when they're imagining an ASR assistant versus imagining a friend or family member. That can sort of close that gap in production as well.
Kat Setzer 14:48
Interesting. Interesting. It sounds like so many exciting opportunities for the research, though.
Michelle Cohn 14:53
Yeah, yeah, definitely.
Kat Setzer 14:55
So were there any limitations to your study? And what are the next steps for your research?
Michelle Cohn 14:59
Yeah, so this was an imagined scenario. So, you know, this isn't how people actually talk to their friends and family members or voice assistants. They don't just imagine talking to them, they do it. And so the next step would be to look at real interactions. And then we're also really interested in how speakers repair errors when they do happen. So what adjustments to speakers make to be better understood when a person or a voice assistant or ASR system misunderstands them. And we also want to look at specific features related to African American English across contexts. So that's something else we're working on. And then also expanding to other language varieties that are misunderstood by voice technology, like L2 speakers. We have a recent paper, led by Jules Vonessen, with Nick Aoki, myself and Dr. Georgia Zellou, coming out of the Phonetics Lab that was actually published in the same special issue on human machine interaction in JASA. It came out yesterday, actually.
Kat Setzer 15:50
Actually, I was gonna say I just saw that one.
Michelle Cohn 15:53
Yeah, where we found that both human and ASR listeners misunderstand L2 English speakers, L1 Mandarin speakers, at a higher rate than L1 Speakers. So not a super surprising finding. But those were also in technology- and human-directed speech adaptations. So it's really expanding on the language varieties that we study.
Kat Setzer 16:15
Do you have any other closing thoughts?
Zion Mengesha 16:17
Oh, yes. We just want to encourage others and all of the listeners to consider examining speech adaptations with technology for African Americans’ speech and other language varieties, as well. I think more work could be, and needs to be, done in this area.
Michelle Cohn 16:31
Yes. 100%.
Kat Setzer 16:33
Yeah, it is really interesting to think about. And actually, we recently did another episode about sort of the importance of teaching a variety of voices and linguistic types in speech science courses. But it's kind of like, from what you're discussing here, that we also need to teach our technology with a variety of speech types. So I can't wait to see where you go with this research. And I wish you the best of luck in your endeavors. Thank you so much for speaking with me today.
Michelle Cohn 16:55
Yeah, thanks so much for having us.
Zion Mengesha 16:57
Thank you so much.
Kat Setzer 17:01
Thank you for tuning into Across Acoustics. If you'd like to hear more interviews from our authors about their research, please Subscribe and find us on your preferred podcast platform.