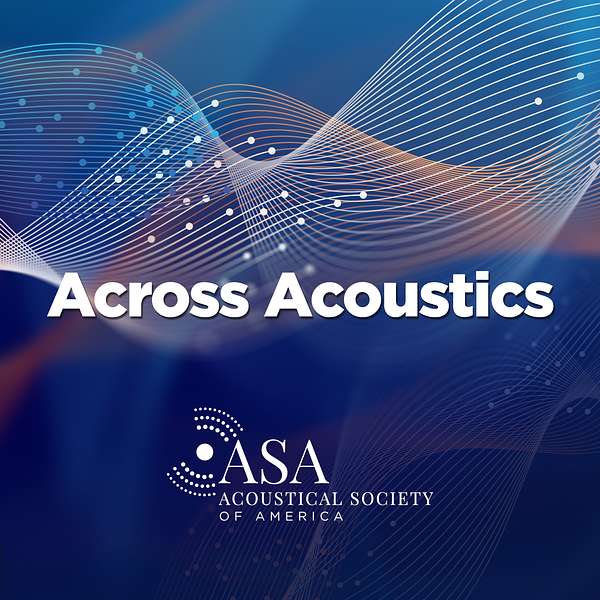
Across Acoustics
Across Acoustics
Student Paper Competition: Emotions of Drums, Acoustic Black Holes, Ocean Noise, and More!
This episode, we talk to a new round of POMA Student Paper Competition winners from the 185th ASA Meeting in Sydney about their exciting research endeavors:
- An analysis of how drums convey emotion
- A method to assess stress caused by vibration in acoustic black holes
- An improved estimator for background noise in underwater signals
- A model to help remove distortion from the sound fields of parametric array loudspeakers
- A numerical study of a little-understood phenomenon in bowed-string instruments
Associated papers:
Zeyu Huang, Wenyi Song, Xiaojuan Ma, and Andrew Brian Horner. "The emotional characteristics of bass drums, snare drums, and disengaged snare drums with different strokes and dynamics." Proc. Mtgs. Acoust. 52, 035005 (2023) https://doi.org/10.1121/2.0001834
Archie Keys and Jordan Cheer. "Experimental measurements of stress in an Acoustic Black Hole using a laser doppler vibrometer." Proc. Mtgs. Acoust. 52, 065003 (2023) https://doi.org/10.1121/2.0001829
David Campos Anchieta and John R. Buck. "Robust power spectral density estimation via a performance-weighted blend of order statistics." Proc. Mtgs. Acoust. 52, 055006 (2023) https://doi.org/10.1121/2.0001849
Wenyao Ma, Jun Yang, and Yunxi Zhu. "Identification of the parametric array loudspeaker system using differential Volterra filter." Proc. Mtgs. Acoust. 52, 055005 (2023) https://doi.org/10.1121/2.0001850
Shodai Tanaka, Hiroshi Kori, and Ayumi Ozawa. "A mathematical study about the sustaining phenomenon of overtone in flageolet harmonics on bowed string instruments." Proc. Mtgs. Acoust. 52, 035006 (2023) https://doi.org/10.1121/2.0001835
Read more from Proceedings of Meetings on Acoustics (POMA).
Learn more about Acoustical Society of America Publications.
Music Credit: Min 2019 by minwbu from Pixabay. https://pixabay.com/?utm_source=link-attribution&utm_medium=referral&utm_campaign=music&utm_content=1022
Kat Setzer 00:06
Welcome to Across Acoustics, the official podcast of the Acoustical Society of America publications office. On this podcast, we will highlight research from our four publications. I'm your host Kat Setzer, editorial associate for the ASA.
Kat Setzer 00:26
We're highlighting another set of POMA student paper winners this episode, this time from 185th meeting that happened this past December in Sydney, Australia. Once again, we've got some exciting new research from various technical committees. First up, we have Zeyu Huang, who will be talking to me about his article, "The emotional characteristics of bass drums, snare drums and disengaged snare drums with different strokes and dynamics." Thanks for taking the time to chat with me today, Zeyu! Congratulations on winning the award. I'm excited to learn more about how drums convey emotions. How are you?
Zeyu Huang 00:56
Thanks for inviting me to this podcast. It's also exciting for me to present my work here to share to a broader audience.
Kat Setzer 01:04
I think a lot of folks will find it interesting. Okay, so first, just tell us about your research background.
Zeyu Huang 01:09
Sure. So I'm studying at the Hong Kong University of Science and Technology, where I'm a third year PhD student. And my main research direction is actually not about acoustics, it's about human-computer interaction under the supervision of Professor Xiaojuan Ma. And my main job is to design, implement, and test interactive systems that kind of solves real world problems and needs. So basically, we are the bridge between computer science theories and real world. But yeah, I also decided to do some musical acoustic research following Professor Andrew Horner because, personally, I'm interested in playing drums. And I also play with my schoolmates in a band. And I also wonder, how can I play drums better in terms of expressing my feelings? And also, I think it is a good chance to build up some theoretical foundations to my human-computer interaction design whenever I need to design acoustic systems or musical systems, I think... yeah, that's how the things are connected between these two fields.
Kat Setzer 02:17
Yeah, it's always fun to hear, like, when you can mix your passions or your interest into your work. So how does perceived emotion relate to pitch usually?
Zeyu Huang 02:28
Yeah, so, this is a question a little bit hard to answer because it really depends on the type of instruments. For example, for brass and bowed stringed instruments, we have found that for example, the happy, the romantic, that kind of positive feeling will generally increase with pitch. So, when the pitch goes up, that kind of emotion will become stronger and for negative feelings like angry and sad perhaps they are generally decreasing with pitch. However, for some other types of instruments, say if we consider human voices, like tenor voice, as instruments as well, then we can find that kind of angry and scary negative feelings also increase with pitch. And for mallet percussion, like marimba, perhaps the instrument itself has some strong characteristics, so most emotions are less significantly affected by pitch.
Kat Setzer 03:26
Okay, okay. So what research has been done so far on how unpitched instruments like drums convey emotion?
Zeyu Huang 03:33
Most research focus on a higher level perspective, for example, an entire groove of drumline, for example, in a rock and roll music, you hear something like [mimics groove]. And that's an entire groove. And that kind of groove will convey a certain emotion. And there are also some work that tries to delve into the components of grooves, say a pattern like, [mimics short groove], that shorter pattern of around one or two measures. But there are less work that focuses on even more micro level thing, like a single drum note, like a snare drum 'ta,' or bass drum 'doomp,' what kind of emotions that these single notes will convey and how these kind of emotions are affected, by the way that we perform those single notes.
Kat Setzer 04:28
Okay, got it. So you're sort of got into this-- what variables were you considering when assessing the drum sound?
Zeyu Huang 04:33
Yeah, so in my work, I use three variables. First is the type of drum sound. For example, I use the bass drum, and also the snare drum, and a special type of snare drum sound called the disengaged snare drum. That is, when we loosen the metal wire beneath the snare drum, it will produce the sound of 'doon' instead of 'ta.' Secondly, we have dynamics, which is how hard we play the drum. And thirdly, the stroke. We can do a single stroke, or we can do a drum roll, that kind of stuff.
Kat Setzer 05:10
That makes a lot of sense. Okay. So how did you assess listener's perceived emotion with relation to these various drum sounds?
Zeyu Huang 05:18
So basically, I choose a lot of drum sounds based on these three variables and then I asked the participants to first rate the location of the sound in terms of valence and arousal plane. So valence and arousal is a common model for emotions where valence is the positivity of the emotion, say happy is high valence and sadis low valence. And arousal is the excitement level of the emotion, for example, happy and angry are high arousal and sad and calm are low arousal. So yeah, I asked them to position each piece of drum sound in the valence-arousal plane. And also, I choose 16 emotions that often appear in other emotional characteristic analysis work. And I asked participants to rate whether each of the emotion is present for each drum sound.
Kat Setzer 06:17
Okay. Yeah, I wonder if I would be able to, like, differentiate emotions if I were listenng to a single drum sound. Anyway... So what is a correlation test? And how did you use it when assessing your listeners' assessments of the drum sounds/
Zeyu Huang 06:30
In my work, I use a correlation test to see whether users' ratings of the presence of each emotion correlates with each other. For example, when a user think that happy is present in this specific drum sound, then is it very likely that, say, excited is also presented? That's the purpose of using the correlation test. So the purpose of doing this test is that I want to first use it as a sanity check. For example, if a user simultaneously rates happy and sad and angry and calm in a single drum sound, that definitely... that's a dirty data. And yeah. The second thing is that we also want to know if there are some nuanced relationship between these emotions. For example, for a specific type of sound, maybe the sad feeling and also the scary feeling are correlated with each other. And that specific type of drum sound cannot distinguish between these two, that's also a finding that we would like to highlight.
Kat Setzer 07:36
Okay, got it. So how else did you analyze the data you received from the surveys?
Zeyu Huang 07:41
I also did a linear regression test. That is the main test that can show whether changing the type of drum from bass drum to snare drum, how will the emotion change? And how will the position of the sound in the valence-arousal plane change? That's basically the two methods that we use: the correlatoon test and also the linear regression.
Kat Setzer 08:05
Okay. Okay. So what did you end up learning about how drums convey emotion?
Zeyu Huang 08:09
So our findings is basically in three parts. First, we realized that compared to bass drums, snare drums are more capable in expressing high-arousal emotions, whether negative or positive, so happy and angry. And normal snare drum sound is especially more capable in expressing this kind of emotion compared to disengaged snare drums. And secondly, I think I've mentioned this point before, like for drum sounds it's hard for them to distinguish between sad emotions, and also scary and terrifying emotions. The third is that since we have this specific technique called drum rolls, which is to produce the kind of consecutive sound, that technique is especially capable in producing agitated emotions, whether negative or positive, so excited or worried. Yep, that's basically the finding.
Kat Setzer 09:12
Okay, yeah, that makes a lot of sense. Yeah. I could definitely imagine that. So what do you see as the next steps for this work?
Zeyu Huang 09:19
So when I present this work at the ASA meeting, a senior scholar remind me that apart from comparing the emotional characteristics of drum sounds between drums, we can do in-depth investigation within one type of drum. For example, drummers in practice will always be figuring out how they can tune their drums to fit a specific music genre. For example, in rock and roll music, perhaps they will make the drum sounds crisper. And for a Lo Fi music and eight bit music, the drum sound will be damper. So as we can see, in practice, we can have different nuanced types or styles for a specific type of drum. And I think it is an interesting direction to study how different styles of one drum can affect the emotional characteristics.
Kat Setzer 10:16
Yeah, sounds like fun. You know, it's funny because it's so easy to think about pitched instruments conveying motion, but it's really interesting to consider how something like a drum sound can also impact how music is perceived emotionally whether it's, like, one type of drum like you're saying or many types of drums. Thank you for shedding light on this new insight into how we perceive music and congrats again on winning this award.
Zeyu Huang 10:37
Thank you. I'm also honored to receive this award.
Kat Setzer 10:42
Next up, we have a student talking about his research in a hot topic in acoustics, the acoustic black hole. Here with me is Archie Keys, who's going to discuss his paper, "Experimental measurements of stress in an acoustic black hole using a Laser Doppler Vibrometer." Thanks for taking time to speak with me today, Archie, and congrats on winning the award. How are you doing?
Archie Keys 11:02
Thank you. Yeah, I'm very well. Thanks for having me on.
Kat Setzer 11:05
Yeah, thanks for being here. So first, just tell us a bit about your research background.
Archie Keys 11:10
I started off doing my undergrad degree at the ISVR in the University of Southampton. I did that in acoustical engineering. And I carried on from there still at the ISVR in the University of Southampton to do my PhD. And my PhD is focusing on reduction of stress in acoustic black holes. And I've got about eight months left until the end of my PhD at this point.
Kat Setzer 11:31
Oh, that's exciting.
Archie Keys 11:33
Yeah.
Kat Setzer 11:35
So the term "acoustic black hole" also sounds very exciting. What is it, though?
Archie Keys 11:40
Yeah, so acoustic black holes are a method for controlling the level of vibration in a structure. But unlike traditional methods, they don't increase the mass of the structure, and they're also quite effective over a wide range of frequencies. So you apply an acoustic black hole as a gradually tapered thickness to the edge or the end of a structure, nd then as the waves come into the acoustic black hole section, the thinner parts of the structure mean the waves travel more slowly. And then the slow waves have a shorter wavelength, which is more easily then absorbed by passive damping treatment. So it sort of ends up extending the low frequency range at which passive damping treatment is effective.
Kat Setzer 12:22
Okay, got it. So how is an acoustic black hole similar to and different from a real black hole? Why do you make this analogy?
Archie Keys 12:30
Yes, so they're actually really very different to a real black hole. But the analogy comes from the kind of effect: Vibrations go into the black hole, but they don't come back out again. So that's predominantly where the analogy comes from. But there's another sort of, I think the reason it was initially named this way is because an ideal use of black hole has zero thickness at the end, which results in a zero wave speed and also a infinite amplitude of vibration at the tip. So that kind of draws parallels with a singularity of density in a sort of space black hole.
Kat Setzer 13:08
Gotcha. Okay. So what are the challenges of using acoustic black holes to attenuate vibration?
Archie Keys 13:15
Okay, so the main challenge, which is what my research focuses on, is the fact that the acoustic blackhole effect results in a large amount of energy being focused into a very thin part of the structure. And what that means is you get very high levels of dynamic stress in the structure, so that stress due to the actual vibrations in the acoustic black hole taper itself. And the problem with that is if you get high levels of dynamic stress over a long period of time, you can get the structure actually failing due to fatigue. So in any kind of applications with a high amplitude excitation, that's really not desirable.
Kat Setzer 13:52
Yeah, understandably. So what are the goals of your research?
Archie Keys 13:57
Yes, so the main goal of my research to start off with is simply to assess the level of dynamic stress in the acoustic blackhole taper, so assess how big of a problem the dynamic stress is based on the amount of force you're putting in. Another sort of goal is to come up with accurate methods to experimentally measure the level of stress. So that's kind of what this paper focuses on, is a method of measuring the dynamic stress in the acoustic black hole taper. And then following from that, another goal of my research is to try to actually reduce the stress. So modify the acoustic black hole profile in some way that reduces the stress but maintains the vibration control performance.
Kat Setzer 14:37
Okay, got it. So why can't traditional methods for calculating stress in a structure be used with acoustic black holes? What do you do instead?
Archie Keys 14:45
Yes, so traditional methods will normally use strain gauges in order to measure the stress in a structure. So the main problems that we have with acoustic black holes with strain gauges is that you're affecting the dynamics of the structure itself when you apply a strain gauge. So the strain gauge itself has mass. So adding mass to a very thin structure, like an acoustic black hole, might become significant. And also, often the adhesive that you use to apply the strain gauge can actually affect the damping as well in the structure. So if you're affecting the mass and the damping of the structure, it may be that the stress measurements you end up with aren't actually accurate to the structure if you didn't have the strain gauge on it. So the way we kind of get around this is to use a laser Doppler vibrometer. So that actually measures the velocity at a single point on the acoustic black hole or any structure. And it doesn't obviously have an effect on the structural dynamics of that structure because it just sends a laser beam onto the structure and reflect it back and uses that to measure velocity. The main problem with this is that we need a way to convert from measurements of velocity to measurements of stress.
Kat Setzer 15:52
Okay, okay. And so that's where we're getting into what you were looking at. What is the numerical model you developed for estimating stress in the acoustic black hole taper, and how did you use it in the study?
Archie Keys 016:04
In terms of the numerical model, we use a two-dimensional finite element model which consists of a uniform beam section, and then an acoustic black hole taper applied to the end so the uniform beam has uniform thickness throughout and then the acoustic black hole taper has a gradually reducing thickness to a very thin but finite thickness at the end. So we then apply a force to the opposite end of the beam, to the end where the acoustic black hole is applied. And we sort of use the resulting displacement field to extract the stress in the acoustic black hole taper.
Kat Setzer 16:42
Ah, okay. Okay. So you then did an experiment to calculate stress in the acoustic Blackhall. How did that work?
Archie Keys 16:50
With the experiments, we kind of wanted to make it as similar as possible to the numerical model. So we use the same structure with a uniform beam with an acoustic black hole termination. We manufactured that from aluminium, which is the same material we use in the numerical model, we applied a force at the same points as in the model, so the opposite end to the acoustic black hole taper, and we applied white noise via a stinger to replicate the free boundary conditions that we applied numerically. We then use the laser Doppler vibrometer to measure velocity across a grid of points on the taper. And that allows us to get the displacement shape at each of the frequencies that we're measuring at. So then we can take that displacement shape and relate it to the stress in the structure. So using Euler Bernoulli beam theory, you can take the second spatial derivative of displacement to calculate the bending stress in a structure, which is supposed to be a uniform beam, but we apply it here to the acoustic black hole taper.
Kat Setzer 17:47
So how did your model into performing?
Archie Keys 17:50
Yes, it performed really quite well in terms of capturing the dynamics of the structure. So the experimental and numerical results line up very well, in terms of the sort of frequencies that the peaks were happening at. The main problem was that the experiments were consistently underestimating the magnitude of the stress in the taper. So it turns out, this is actually to do with the assumption that we have an Euler Bernoulli beam. But an acoustic black hole is not an Euler Bernoulli beam. So it's going to change in thickness, whereas an Euler Bernoulli beam has a consistent thickness. It's also a lot thinner in sections than an Euler Bernoulli beam mightt allow for, it has higher damping, that kind of thing.
Kat Setzer 18:31
Okay, yeah, that makes sense. What was the most interesting or exciting aspect of this research for you?
Archie Keys 18:38
I think, for me, the most interesting part is trying to identify the sources of error that we had, between, as I've said, the experimental model and the numerical model. So the way we use Euler Bernoulli beam theory meant that we had these errors. And when we use the same methods numerically and experimentally, we didn't have the errors. So the errors weren't coming from, say, noise you might get experimentally, they were actually coming from the calculation we were forming. So even if we took the experimental measurements perfectly, and ideally with no noise, we would still be having the same errors.
Kat Setzer 19:10
Right, right. So this is a good segue for what's the next step in your research?
Archie Keys 19:15
Yeah, yeah. So following on from this paper, we did a lot of work identifying the sources of error. And yeah, we've sort of come up with a few reasons. It's mostly to do with the thickness of the acoustic black hole taper and the level of damping. So we know that the error is kind of inherent in the method we were using. So we're also developing a new method potentially for measuring the stress using strain gauges, which obviously I said earlier, has different problems associated with it. But it's possible that those problems are less impactful than the issues we were having by assuming an Euler Bernoulli beam. So the importance here is if we can get an accurate measurement of stress in an acoustic black hole, it allows us to properly assess the level of stress in the acoustic black hole so we can properly see if it actually is useful for applications where an excitation might be high in magnitude. And we're developing a modified profile as I mentioned earlier. We want to be able to prove that the modified profile actually reduces the stress in the structure compared to the conventional acoustic black hole.
Kat Setzer 20:17
Wow, okay, well, I will keep my fingers crossed for you that these different options work out. I'm not gonna lie, I've always wondered what an acoustic black hole was, and it was so really fun to, like, learn about them and some of their limitations by talking to you. It's also very cool that you've been able to, maybe not improve on current techniques, but you know, understand what's going on with these techniques and how to fix them.
Archie Keys 20:41
Yes, yeah.
Kat Setzer 20:42
Yeah! Thank you again for taking the time to speak with me today and congrats on winning the award.
Archie Keys 20:47
Thanks very much.
Kat Setzer 20:49
Yeah, you're welcome!
Kat Setzer 20:52
Next up, I'll be talking to David Campos Anchieta about his article, "Robust power spectral density estimation via a performance-weighted blend of order statistics." Congrats on winning the award, David, and thanks for speaking with me. How are you?
David Campos Anchieta 21:05
Well, thank you. Oh, well, I'm fine. Glad I got the award.
Kat Setzer 21:10
Yay! That's good. So first, just tell us a bit about your research background.
David Campos Anchieta 21:14
So yeah, I started my grad school almost six years ago at the University of Massachusetts Dartmouth. At first I did a lot of array signal processing and adaptive beamforming. And when I started my PhD, I took some of the ideas that I developed in the work with adaptive beamforming and took it to spectral estimation, basically, this kind of problem of estimating the PSDs using order statistics.
Kat Setzer 21:41
Okay. So what is background noise power spectral density, and why are accurate estimates of it important in underwater acoustic signal processing?
David Campos Anchieta 21:50
Okay, yes, those are a few concepts. So firstly, the power spectral density, or the PSD as we like to say, is kind of like a function that tells you how loud each frequency is in a piece of signal, in our cases, audio, more specifically, underwater acoustic recordings. Okay? It's kind of like, making an analogy with music, it's kind of like, you know, you're counting, you take a piece of piano music and you count every time a key is pressed, and you do some time average, something like that. And for the background noise, PSD, basically, you know, the background noise in any environment, but I guess in the underwater environment, it can carry some information about some conditions in the environment, such as what we in our case, in our project, we're looking mostly for surface wind and rain. So when it's rainy, or when it's windy out, it can change the shape of the power spectral density of the background noise in a way. So yeah, that's basically why we want to estimate the PSD of the background noise. So we can infer information about the environment where the sound was recorded.
Kat Setzer 23:01
Ah, okay. Okay, that makes sense. So in your paper, you talk about a couple different methods for estimating power spectral density for underwater noise. What are those methods?
David Campos Anchieta 23:12
Yes, we talk first about the Welch method, which is a classic method, it was developed in the 1960s, which basically, instead of computing the power spectral density, like for, you know, a whole piece of audio where you want to estimate the PSD, you break this audio into several smaller pieces that have some overlap between them. And you compute the PSD of each of those smaller pieces, and you do an average of each of those PSDs to get your PSD estimate. And this reduces the variance of the PSD, has some advantages over computing the whole signal. But it may be very sensitive to what we call loud transients, or outliers in data, in a sense, because of the sample mean, or the averaging, it can be very distorted by outliers in data. I like to make the analogy, like what if you want to survey the average income of a group of people in a classroom, but you don't realize that Jeff Bezos was sitting in a classroom, so you would have a much higher salary that doesn't really represent the population that you have.
Kat Setzer 24:21
Right. It's kind of like the mean, or average versus the median?
David Campos Anchieta 24:24
Yes.
Kat Setzer 24:25
And what those mean... right. Got it.
David Campos Anchieta 24:27
So yeah, the second spectral estimators that I mentioned in the paper was the Schwock andAbadi Welch percentile estimator, which tries to solve this problem of sensitivity to outliers, or the loud transients, by instead of doing an average of the PSDs of the smaller chunks of data, they do what we call order statistics filtering. So order statistics, in a sense, it's like when you have a set of data, and you sort them by order of magnitude. And you can pick like, if you pick the one that is going in the middle, you can, it's what we call the median, you can take the maximum and you can take the minimum, but Shwock and Abadi kind of generalize this to do an estimate of where you can pick any order statistics you want, depending on how frequent your outliers are in your data. And you can do an spectral estimation that is more robust against those loud transients or outliers in data.
Kat Setzer 25:21
Okay. Okay. So what are the limitations for these two estimators?
David Campos Anchieta 25:25
Well, for the Welch method, well, the limitation, right, it was the sensitivity to outliers. The Schwock and Abadi kind of solves the sensitivity to outliers problem, but it brings another limitation, it brings another problem, which is that we have to pick the order statistics that we actually want to use for our estimators whether, I mean, it could be the median, it could be something closer to the maximum or something close to the minimum of the power spectral density for each frequency. And you have to pick that basically based on some intuition or some information that you have about your data. So, well, if your outliers are more frequent, you may want to pick a lower order statistics. And if your outliers are, are less frequent, you may want to pick a higher one because the higher order statistics also tend to have a lower variance, but they are more subject to the loud transients and the lower order statistics, they have a higher variance, but they are more robust against the loud transients. So there is this trade off. And also, there is a situation where throughout your data, those outliers may be more or less frequent. And you may need to adapt, you may need to change the order statistics that you use to adapt to those changes and keep the variance of the estimator as low as possible. So you have a more precise estimate.
Kat Setzer 26:45
Okay, so you propose an updated estimate or the universal SAWP. How did you develop this estimator, and how does it differ on the previous estimators?
David Campos Anchieta 26:54
Yes, we produced this universal SAWP, which basically borrows the technique from the universal linear prediction algorithm that was proposed by Singer and Feder in the in a paper like, I think from the 90s. And this algorithm has been used in some problems where this problem of model order selection appears, in essence. So in our case, we need to pick an order statistic for the Shwock and Abadi Welch percentile estimator. But what if instead of picking one, when we are processing data online, or like in real time, we assess the performance of each of those estimators, and we make some kind of a blend, a weighted sum of those estimators that perform better over the past few time iterations. When you're processing the data, I can make an analogy, it's kind of like, you know, when you walk into an ice cream shop, and you cannot really decide which flavor you want to pick. And you may ask whoever is serving the ice cream to give you like a half a scoop of this and 30% of a scoop of that flavor. Yes, kind of like this one. But with order statistics. Tat may not be a very good idea to do with ice cream, but in this kind of engineering problems, sometimes it works. Yeah, basically, we do a blend of the order statistics estimators that are working better for the data that we have based on their sample variance over the past few iterations.
Kat Setzer 28:22
Okay. So how did you assess the feasibility of the universal SAWP?
David Campos Anchieta 28:26
In this specific paper, we just did some simulations with synthetic data. We tried to make data that mimics the situation of trying to estimate the power spectral density of background noise in an environment that has occasional very loud outliers. We first did, like, one trial with, we tried to make some data in which you know, every few time iterations, the occurrence of the outlier is increases by 2% points. So it starts with zero, then 2%, and 4%, and so on. So we can test how the universal algorithms adapt to those changes in the occurrence of the outliers. And the result is that well, at least one trial was really good. The estimator would quickly shift its weights to lower order statistics as the occurrence of the outliers increased. And when you see the graphs in the paper, also the squared error of the estimator was also lower than any of the fixed-rank estimators. We also did some Monte Carlo trials. So we did several trials. So we could assess, like, the bias and the variance and the mean squared error in general. And even though the universal estimator has maybe a little bit of a bigger bias than some of the fixed order statistics, it kept a lower variance than any of the fixed order statistics estimators in it also kept a lower mean squared error than any of them. So overall, the estimator was able to quickly adapt to increasing occurrence of outliers and was able to also perform better as an estimator of the background noise bar.
Kat Setzer 30:00
That's exciting. What are the next steps for this research?
David Campos Anchieta 30:03
In this, basically, we could test it on some real data, like some, you know, hydrophone array data to see and maybe plug into a bigger algorithm like in a system that can detect or estimate rain and winds and see if it helps get a more accurate estimation or detection.
Kat Setzer 30:22
So what was the most surprising or exciting aspect of this research for you?
David Campos Anchieta 30:26
I guess the most surprising is how consistently the universal algorithm performed better than the fixed rank. I mean, it's really nice to see on the graph that it consistently kept a lower error, and it kept a lower variance and mean squared error than all of them. I didn't need to dig in too much with the parameters to achieve that kind of result. That was very surprising, and it was also the most exciting part
Kat Setzer 30:51
Yeah it's always nice when you're like, "Oh this thing that I'm doing actually works pretty well!" Right?
David Campos Anchieta 30:56
Yes
Kat Setzer 30:57
Well, thank you again for taking the time to speak with me today and congratulations on the award. And I wish you the best of luck in your future research.
David Campos Anchieta 31:04
You're welcome. Thank you.
Kat Setzer 31:07
Next up, I'll be talking to Wenyao Ma about her article, "Identification of the parametric array loudspeaker system using differential Volterra filter." Thanks for taking the time to speak with me today, Wenyao, and congrats on your award. How are you doing?
Wenyao Ma 31:21
I'm great. Thank you for the invite.
Kat Setzer 31:25
First, tell us a bit about your research background.
Wenyao Ma 31:28
Well, my name is Wenyao Ma. Now I am studying for my PhD at the Institute of Acoustics, Chinese Academy of Science and I major in audio signal processing. To be specific, it is concerned with methods in identification of nonlinear effect in loudspeaker, inverse problems of nonlinear system, and compensation for nonlinear distortion. The parametric array loudspeaker is such a nonlinear system.
Kat Setzer 32:02
Okay, so what are parametric array loudspeakers?
Wenyao Ma 32:07
So the parametric era loudspeaker RPL for church is a loudspeaker that uses ultrasonic waves to create a highly directional audio sound field, which is commonly used in settings like museums, exhibitions and public places where creating a personal audio zone is essential. To realize it, audible sound information, for example music and speech, is modulated onto the ulrasonic carrier waves. As the modulated waves are input to an ultrasonic transducer and then emitted into the air, they interact nonlinearly with the medium. This interaction causes the ultrasonic waves to demodulate and generate audible sound waves contain initial audible sound information. For example, when the emitted ultrasonic waves contains two frequencies at 40 and 41 kHz, the new frequencies at 1 kHz and 81 kHz will be created according to the nonlinear interaction. The 1 kHz frequency, or to be more general, the difference frequency is human audible and of interest to us. Thus, the audible sounds are generated and inherit a high direction from the ultrasonic waves.
Kat Setzer 33:40
Okay, that's pretty cool. So why do folks use these more complicated speakers instead of normal ones?
Wenyao Ma 33:46
Yeah, it is a good problem. If we want to obtain the focused sound using the conventional loudspeakers, we have to resort to array. However, the smaller the sound zone is required, the larger the speaker array should be. Fortunately, the PAL can do better with a smaller size because ultrasonic waves have short wavelengths, and this allows them to maintain a narrow beam over a long distance, which is like an spotlight for sound with a narrow sound beam. It is real magical.
Kat Setzer 34:22
It does sound pretty magical, doesn't it? So what is nonlinear distortion ,and how does it impact the sound fields generated by parametric array loudspeakers?
Wenyao Ma 34:33
Because the system works relying on nonlinear effect, the distortion is also generated together with the desired audio signal. The distortion usually contains the harmonic and intermodulated components. For example, the PAL emits a carrier wave at 40 kHz and sidebands at 41 and 43 kHz. We hope the sidebands only make nonlinear interaction with the carrier, to produce the desired audible tone at 1 kHz and 3 kHz. However, the sidebands’ self will interact with each other and produce an undesired 2 kHz tone. This process happens at the same time with the desired part. In the case of wideband signals, such as music and speech, distortion is significant and requires preprocessing techniques applied on the signals before they are emitted into the air. It is essentially an inverse problem. So the distortion reduction is a challenging task.
Kat Setzer 35:45
Okay, that's really interesting. What methods have previously been used to model the sound fields of parametric array loudspeakers, and what are the limitations of these methods?
Wenyao Ma 35:55
The mainstream methods can be divided into two parts. The first part is the frequency-domain method, which is obtained by solving wave equations. They are usually calculated by numerical integrals. So the computation is time-consuming. Another part contains nonlinear adaptive filter methods based on the nonlinear model, like the well-known Volterra filter. However, a generic response is difficult to identify. That is to say, the learnt response varies across different training data. Actually, the Volterra filter is usually introduced heuristically to do the PAL identification task. It is reasonable to question that Volterra filter model is not the most suitable structure for PAL system.
Kat Setzer 36:51
Okay, so, what is the Volterra filter model?
Wenyao Ma 36:54
The Volterra filter is a nonlinear extension of the classical linear filter. It takes into both linear and nonlinear relationships between the input and output signals, and it is often used to model a nonlinear system.
Kat Setzer 37:12
Okay, got it. What were the goals for the model you propose?
Wenyao Ma 37:16
My first goal is to propose a simple and accurate model to describe the audible sound generated by the PAL in the field close to the transducer surface. Our final purpose is to create a personal audio zone, and the distortion reduction is one of the most important parts in the system design and optimization. As far as I know, the inverse system design is very complex, especially in nonlinear system. So we hope the modeling to be as simple as possible, and this will help a lot in the inverse system design later.
Kat Setzer 37:56
Okay, got it. How did you build upon the Volterra filter for your model?
Wenyao Ma 38:02
Our proposed model reshape the input signals into a version that conforms to the physical process of PAL. Moreover, if we expand the reshaped input, it has a similar form with conventional Volterra filter but our proposed model is simpler.
Kat Setzer 38:23
Okay, so how did you end up testing your model?
Wenyao Ma 38:27
The axial region is the focus of most studies because it covers the major energy of the sound beam. Currently, our primary focus is on modeling the on-axis sound field. So during this experiment, we fed the cosine tune with multiple frequencies into the emitter, and recorded the audible sound generated on the axis. Then, we compared the predicted results from our proposed model with the measured audible sound and the predicted results from other mainstream models. The convergence speed of the model is also tested compared with existing models. It is considering the fact that, the closer the model structure is to the real system, the faster the response is learnt.
Kat Setzer 39:23
How did your model perform?
Wenyao Ma 39:24
Our proposed model spent less time to converge to a stable response. The obtained response using our proposed model predicted more accurate sound pressure level than existing Volterra filter methods.
Kat Setzer 39:40
Well, that’s pretty exciting. What are the next steps for this research?
Wenyao Ma 39:43
We are going on the algorithm design for the distortion reduction. We do the identification work first to pave the way for this target.
Kat Setzer 39:53
So what was the most interesting or exciting part of this research for you?
Wenyao Ma 39:56
I like the experiment process most. Because I can truly feel the narrow sound beam it produces, and the sensation of the sound source surrounding me as if it was right in front of me. I believe this technology will be loved and welcomed in the future.
Kat Setzer 40:14
Well, thanks again for taking the time to speak with me today. It was really fun getting to learn about the speakers that I guess I've encountered so many times in museums and exhibits and such. Congrats again on your award and your future research.
Wenyao Ma 40:26
Thanks for having me on the podcast. It was my pleasure to have the opportunity to talk about my work here. Thank you for the wishes.
Kat Setzer 40:36
Last but not least, I'm talking to Shodai Tanaka about another musical acoustics paper, A mathematical study about the sustaining phenomenon of overtone in flageolet harmonics on bowed stringed instruments." Thanks for taking the time to speak with me today, Shodai, and congrats on winning the award. How are you?
Shodai Tanaka 40:53
Yes, I'm doing good. I'm very honored to join this podcast.
Kat Setzer 40:57
You're more than welcome. So first, tell us a bit about your research background.
Shodai Tanaka 41:01
So my name is Shodai Tanaka. I'm currently 19 years old, and I was a high school student in Sapporo, Japan, when I submitted the paper. I have been a violin lover since I was four years old, and I'm also a classical violin player. The reason why I love the violin is because of its beautiful sound within its complexity. I was curious about why such complex sounds came out from the violin and the mechanism behind them. This triggered me to learn about the physics of violins. I also learned the physics of the violin by reading through ASA articles, and I was very surprised to learn that there are so many acousticians who pursue the complexity of the musical instruments and how beautiful their analyses are.
Shodai Tanaka 41:54
I started this research when I entered high school. I was supported by UTokyoGSC, a program organized by the University of Tokyo to promote scientific research among high school students, where I met Professor Hiroshi Kori and Dr. Ayumi Ozawa, who are the co authors of this research. I would like to thank them for their continuing support and interesting discussions. We have been working on this program for two years.
Kat Setzer 42:26
Well, that's pretty impressive. I'm not sure I was up to this level of research when I was in high school. So what are flageleot harmonics?
Shodai Tanaka 42:34
So, flageolet harmonics is a playing technique for stringed instruments, in which a player lightly touches a nodal point of the string with their finger to produce so called overtones. For example, on the G string of the violin, when you bow the string, you hear this [violin playing note]. Next, if you lightly touch the half point of the string, you hear sound musically one octave higher than the previous sound. Namely, it has a frequency that is twice its fundamental frequency. It sounds like this [violin playing note]. This sound and technique are called graduate harmonics. Flageolet harmonics are widely used in various musical pieces, especially those played by virtuoso violinists. It looks simply when played by a virtuoso, but when analyzed mathematically, it is quite a complex problem.
Kat Setzer 43:40
Yeah, I could see how that would be pretty complex. What is the harmonic sustaining phenomenon and why does it seem to occur?
Shodai Tanaka 43:47
In my daily viioolin practice, I have observed an interesting phenomenon related to flageolet harmonics. I attempted to remove the finger from the string after playing harmonics, while I kept bowing. It was at time that I found interesting phenomenon, that the sound of overtone sustained for a short time, even after the finger is removed from the string. I named this phenomenon the harmonic sustaining phenomenon. More interestingly, this sustained time of harmonic sound after removing the finger depends on the bowing parameters, such as bow speed, bow force, and bow position. Most violin players recognize this phenomenon and feel that it is normal. However, we don't fully understand its mechanism yet. The harmonic sustaining phenomenon has also been mentioned in several papers, but the investigation of this phenomenon is still limited. Therefore, we aimed to understand the mechanism of this phenomenon by using mathematical approaches.
Kat Setzer 44:58
Okay, so why is it useful to know the sustaining time?
Shodai Tanaka 45:02
Some violinists often make effective use of this phenomenon in their music when the movements of the left hand fingers are very busy. Proper use of this phenomenon allows the player to prepare for the fingering of the next sound by the moving the finger earlier, while the previous harmonic sound is still sustaining. In this case, the player wants to sustain time to be as long as possible to ensure longer preparation time for the next sound. Therefore, understanding the parameter dependency of the sustaining time could contribute to providing players with tips for controlling the sustaining time.
Kat Setzer 45:44
Okay, yeah, that totally makes sense. So you wanted to create a mathematical model to help understand the sustaining phenomenon. How did you develop this model?
Shodai Tanaka 45:53
From empirical observations, we first assumed that the string, bow, and finger are at least required to reproduce the phenomenon. We constructed the mathematical model based on previous research on both bowed stringed instruments and flageolet harmonics. First is a mathematical model of the bowed string instruments. Here the string is expressed by the wave equation of the string, and the bow is expressed by a nonlinear frictional force applied at only one point. Second is a mathematical model of the flageolet harmonics on plucked string instruments. Here, the string is expressed by the wave equation of the string and the finger is expressed by a damping force applied at only one point. Then, we combined those different models for bowed string instruments and flageolet harmonics to create the model for flageolet harmonics on bowed stringed instruments. In addition, we need to express the removal of the finger to create the phenomenon. Thus, we use Heaviside's step function to express the instantaneous removal of the finger. We were then able to construct a simple model for harmonic sustaining phenomenon.
Kat Setzer 47:15
Okay, so then how did you test that the model reproduces the sustaining phenomenon?
Shodai Tanaka 47:20
So, we analyzed the model using numerical simulations. We have obtained the time series of the peak values of force acting on the bridge for fundamental tone and overtone. We have obtained the force acting on the bridge. Because this is a main factor that contributes to the actual sound we hear. Compared to simulation results of the standard playing, in which a finger is removed for all time, we observe that the evolution of fundamental tone is considerably slow in the case of simulation results with the finger is removed after the flageolet harmonics. Therefore, the harmonic sustained impediment and it's successfully reproduced in our model.
Kat Setzer 48:07
How did you assess which parameters affect sustaining time?
Shodai Tanaka 48:10
We define the sustaining time as the time needed for the fundamental tone to lead the over tone after the finger removal to investigate the parameter dependence. We first investigated the bowing parameter dependency, including bow speed, bow force, and bow position. We found that the sustain time is longer with the higher bow speed, the smaller bow force, and the bow position closer to the bridge. These results are consistent with the empirical observations. We also obtained the parameter dependency of sustainimg time for string characteristics, including string tension, string length, linear density, and frictional coefficients for each.
Kat Setzer 48:57
That's really cool. So what did you end up learning about the harmonic sustaining phenomenon with your model?
Shodai Tanaka 49:03
The violin produces a beautiful sound, but it can also produce a horrible sound that is unbearable to listen to. For example, if the bow forced applied to the string is very, very high, it will produce a raucous or sound like this. Be careful audience. I will make really noisey sound. [Violin screeches] And if the bow force applied to the string is low, it will produce the surface sound like this [violin note]. Interestingly, it is understood that there are clear boundaries to create those sounds in the parameters between them. According to the previous studies of bowed stringed instruments, these boundaries characterize the predictability of bowed stringed instruments. And this research we ended up learning that the harmonics sustaining phenomenon its related to this playability of bowed string instrument. The estimated formula in this research tells us that the sustain time will be longer as the parameter it's closer to the boundary for producing surface sound. And in contrast, sustaining time will be shorter as the parameter is closer to the boundary for producing raucous sound. These facts indicate the relationship between sustaining time and the playability of bowed string instruments.
Kat Setzer 50:41
That is super cool. So what are the next steps for this research?
Shodai Tanaka 50:46
So this research is based on the simulation results. Thus, we still need experimental verification of the results. In addition, in this research, we investigated the harmonic sustaining phenomenon for second harmonics, which the player touches the half point of the string, for initial investigation. We are also interested in the analysis of the harmonic sustaining phenomenon for higher harmonics.
Kat Setzer 51:15
Yeah, those do sound really like interesting things to look into. So, what was the most exciting or interesting aspect of this research for you?
Shodai Tanaka 51:24
It is very interesting that the qualitative trend of the parameter dependency of sustaining time is reproduced using our simple mathematical model. In this research, it is also found that the parameter dependency of sustained time follows a power law. The power rule is a relationship that can be expressed by y equals ax to the power of b, where a and b are the constants. In our analysis, we found that the sustaining time dependence on parameters suggests bow speed, force, and so on all follow the power law relationship against the sustaining time. This is an intriguing result, we aim to derive analytically the results about the power law dependency of sustaining time to understand the origin of its power law dependency.
Shodai Tanaka 52:18
As a violin player, it is very interesting to know about the mechanism behind the violin playing. I want to continue pursuing research about uncovering the experiential wisdom that the virtuoso uses in controlling the bowed string instruments.
Kat Setzer 52:36
Thanks for telling us a bit more about this really fascinating phenomenon. This must have been a really fun research project. I wish you the best of luck on your future studies, and congratulations again.
Shodai Tanaka 42:46
Thank you so much.
Kat Setzer 52:51
Thank you for tuning into Across Acoustics. If you'd like to hear more interviews from our authors about their research, please subscribe and find us on your preferred podcast platform.