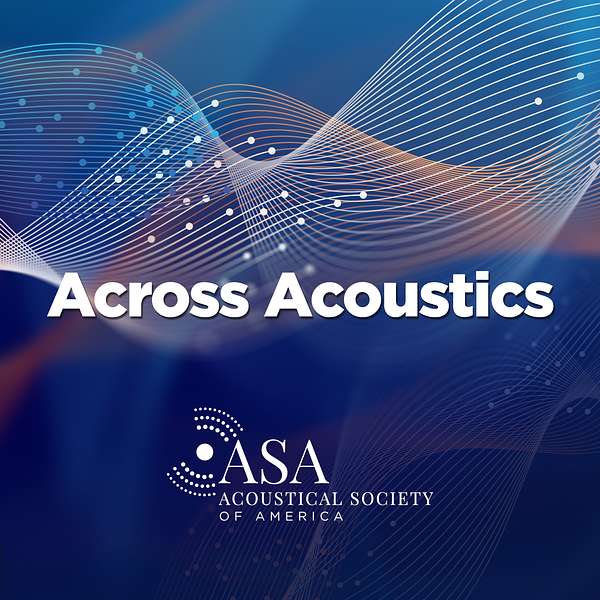
Across Acoustics
Across Acoustics
Deep Faking Room Impulse Responses
It's not always feasible to measure the sound field generated by an acoustic source; instead, scientists have to model to come up with a best guess for the missing pieces of the sound field. In this episode, we talk to Efren Fernandez-Grande and Xenofon Karakonstantis (Technical University of Denmark) about their new machine learning method to reconstruct sound fields.
Associated paper: Efren Fernandez-Grande, Xenofon Karakonstantis, Diego Caviedes-Nozal, and Peter Gerstoft. "Generative models for sound field reconstruction" J. Acoust. Soc. Am. 153, 1179-1190 (2023) https://doi.org/10.1121/10.0016896 .
Read more from The Journal of the Acoustical Society of America (JASA).
Learn more about Acoustical Society of America Publications.
Music Credit: Min 2019 by minwbu from Pixabay.
Kat Setzer 00:06
Welcome to Across Acoustics, the official podcast of the Acoustical Society of America's publications office. On this podcast, we will highlight research from our four publications. I'm your host, Kat Setzer, editorial associate for the ASA.
Kat Setzer 00:25
Today I'm talking with Efren Fernandez-Grande and Xenofon Karakonstantis about their article, Generative models for sound field reconstruction," which appeared in the February 2023 issue of JASA. Thanks for taking the time to speak with me today. How are you guys?
Efren Fernandez-Grande 00:39
We're great. Thank you very much for having us. It's a pleasure.
Xenofon Karakonstantis 00:42
Thanks on my part, too. It's great to be here.
Kat Setzer 00:45
Thank you for being here. So first, tell us a bit about your research backgrounds.
Efren Fernandez-Grande 00:49
Yeah, well, my name is Efren Fernandez. I am an Associate Professor at the Technical University of Denmark, and also a Fellow of the of the society, as well as Associate Editor for JASA. My research focus has been naturally expanding over the years. But in general lines, I would say it's concerned with sound-field analysis and experimental methods in acoustics, acoustic holography, and array signal processing... So I'd say those are the main lines.
Xenofon Karakonstantis 01:15
So yeah, I'm Xenofon Karakonstantis, a fairly hefty name there. But I'm a PhD at the Technical University of Denmark, and my background primarily lies in acoustic signal processing and machine learning. And my research throughout my PhD has been on data-driven methods for sound field reconstruction.
Kat Setzer 01:33
Awesome. So this study has to do with soundfield reconstruction. Can you give us a bit of background about what sound field reconstruction is and how it's used?
Efren Fernandez-Grande 01:43
Yeah. Sound field reconstruction, we could say that it aims at estimating or capturing a sound field in space from a set of measurements. So typically, we would use a sensor array or distributed measurements across space. So to give an example, say that you would like to capture and analyze the sound field radiated by some acoustic source, an engine or a machine or a musical instrument, because you want to better understand how it radiates to produce a sound. So then you measure the sound pressure generated by it a few different positions in space. And then from those measurements, you try to reconstruct the sound field. So you try to essentially estimate what the sound field is anywhere around the source-- so also positions that you have not measured. And with that, you get pressure, velocity and intensity. So this principle is quite powerful and helpful to understand complex sound fields. So therefore, it is applied to, often, sound radiation, noise identification, room acoustics, audio capture, material characterization, and a fairly long list of applications.
Kat Setzer 02:50
What are some kinds of sound fields that you would be reconstructing?
Efren Fernandez-Grande 02:54
So often, you would aim to capture sound fields that are difficult to model or that are complex in the way that it's easier to directly observe them. So let's say if you have, you know, like a room that has some intricate geometry, or maybe some source that it's fairly, well, hard to model or to understand, then it's actually easier to just observe directly the sample and look at its properties.
Kat Setzer 03:21
Okay. Okay. So what are the current challenges with acoustic array processing used in sound field reconstruction?
Efren Fernandez-Grande 03:27
Yeah, so I would say that maybe one of the largest challenges, which is, well, common to several areas for acoustics, is that it's essentially the human audible range, right? Like, because we hear from 20 Hertz to 20 kilohertz, this corresponds to wavelengths that are as large as 17 meters in air-- so that's as large as a multi-story building-- and also wavelengths that are as small as 17 millimeters, which is, you know, essentially like the diameter of a button or a coin. So this brings about a huge challenge because at low frequencies, then, you should measure over long distances or long apertures to capture this long wavelength; then at high frequencies, you would need many sensors that are placed very close together, just millimeters apart. Right? So this poses a very unavoidable challenge or problem, but it's not just, the practical aspect of the measurement, but also how data or how those different types of data, low and high frequencies can be processed, right? There is no one-size-fits-all because the processing at low, mid, high frequencies can vary a lot.
Kat Setzer 04:41
Okay, got it. So why can't you just add a bunch of transducers to your microphone array?
Efren Fernandez-Grande 04:46
Yeah, that's a really good question. I mean, adding more transducers will certainly improve the spatial resolution, and that always helps, but it does come at a price, so... And that's both figuratively but also literally, because it is expensive to add those transducers. And it's also cumbersome to manage that because you not only have the actual transducers, you also have data acquisition, array hardware, and you know, like the structure of the array and so on. And also synchronization, computation issues, and so on. So it becomes too cumbersome and expensive.
Efren Fernandez-Grande 05:24
Also, another thing is that if you have many sensors, especially at high frequencies, many sensors that are placed together very closely, that will also disturb the sound field. So, you know, that dense group of sensors will scatter the sound field and be fairly invasive, right?
Kat Setzer 05:40
Okay.
Efren Fernandez-Grande 05:40
Now, if you look at the trend of things as well, like, it's clear that sensors are becoming cheaper and smaller, especially if you look at MEMS and so on. So you could say, what if, you know, say, in the future, you can have arrays that have very tiny sensors that do not scatter sound and are cheap, right? There, the challenge would be that, if you have, say, for example, to take an illustrative example: So you have a room, and you want to measure the sound field over an area, say, where potentially the head of the listener might be placed. And you'll have all your sensors that are placed very close together to capture wavelengths that are as small as say, 17 millimeters or a few centimeters, right? So you have these very detailed measurements. But then something in the room is moved, say a chair or some object in the room is moved, then the sound field can change drastically, or at least the pressure that you measure can change drastically. So then that raises the question, is it worth measuring everything, everywhere? Right? It would seem that it's much more appropriate to instead measure some statistical properties that are sort of representative of the sound field at those high frequencies rather than going for a deterministic, very fine and precise approach. So essentially, to, you know, make this long response a bit a bit more condensed, there are both the, I guess, the cost challenges, but also, fundamentally, it could make sense to add transducers, but only to a certain point.
Kat Setzer 07:12
Right. Right. That makes a lot of sense. Yeah. It sounds like there are so many factors that you would have to predict in some cases, like you said, if there's a chair that moves or something like that, then it's just not feasible to just sit there putting in microphones every single spot.
Efren Fernandez-Grande 07:27
Yeah, exactly. And then the question, is that like, is the sound field fundamentally different? Probably not. If you just move your coffee cup in the room, then wavelengths that are that small might change, but you could say it's essentially the same sound field, right? So...
Kat Setzer 07:42
Right, right.
Efren Fernandez-Grande 07:42
It's a very good question.
Kat Setzer 07:43
So what are other methods used to extend the frequency range of a sound field measurement? And what are their limitations?
Efren Fernandez-Grande 07:51
I'd say that perhaps the most significant one is, or are, sparse signal reconstruction techniques, such as compressed sensing, which under certain conditions enables us to overcome the classical high-frequency limits from measuring or sampling a signal. So this approach has been exploited for maybe a few decades in audio compression, source localization, also acoustical holography, reconstruction and reproduction. So there's a few domains where this has been used. Now, the issue with it is that in many acoustical problems, the conditions required for the technique to work are not met, right? So, for instance, in scenarios where there is multiple waves that are present simultaneously, then the sound field cannot be considered to be sparse, which is one of the requirements of these techniques. So then it's practically a challenge, right? And this is the case, for instance, in rooms where you have multiple waves that are present at the same time.
Kat Setzer 08:52
Got it. Okay, so in this study you're exploring an alternative approach. Can you explain what the approach is and how it's different from these other methods that we were just discussing?
Xenofon Karakonstantis 09:01
So yeah, I guess allegorically speaking, imagine if you have like a puzzle that's missing several pieces that represent the sound field, and specifically the ones that complete the pictures detail in terms of, like, frequency. So in our study, we use a particular kind of neural network architecture known as generative adversarial networks. And these helped us to fill in those missing pieces in the sound fields or this puzzle, for example, and particularly the high-frequency components of this puzzle. So unlike other methods, which are a bit like trying to guess the missing puzzle pieces, based on the box's picture, like physical representation of a sound field, then GANS, or generative adversarial networks, work by learning from what's already there, like the data, and particularly the lower-frequency bandwidth. So those parts of the sound field is what we use to actually extrapolate to higher frequencies. And then use this knowledge to recreate essentially what's missing. So to answer the pot, that's why it's preferable. The advantage of using generative adversarial networks lies in their ability to generate high-quality and high-frequency components without explicit modeling, and this makes this method efficient and straightforward.
Kat Setzer 10:19
Okay. Okay, so can you explain a little bit more what generative adversarial networks are? And how would they be able to help with reconstructing the information you don't know about in a given sound field?
Xenofon Karakonstantis 10:32
Generative adversarial networks essentially consists of two neural networks, a generator and a discriminator. That's what they've been coined. This generator network, it aims to produce realistic samples according to the statistics of some target data. While the discriminator learns to distinguish between real data and generated samples from the generator network. Each time the generator synthesizes a sample, it's given feedback by the discriminator in order to improve. This back and forth is known as adversarial training. So the ultimate goal of the generator is to reach a certain equilibrium over time where the discriminator can no longer make a distinction between the generated and the real samples. So in our particular scenario, the generator's challenge is to recreate the high frequencies of a sound field. And each time the generator produces a sample, the discriminator examines it closely to determine if the sample could actually be a real sound field, or just the clever fake sound field. And once the training is complete, the generator has to learn to generate these high-frequency sound fields, while including the high frequencies of the sound fields. And this is a cool attribute, because especially when facing the challenges that have been mentioned before, it allows us to enhance the reconstructed sound fields with all the details of a sound field before it's measured, for example, in a room.
Kat Setzer 12:03
That's really interesting. So it's kind of like, like ChatGPT, which is everywhere right now. Folks create these descriptions or whatever, write something, and then you look at it and you say, Is this really correct in any way possible? Or is it you know, very incorrect and making up stuff that's nonsense? And so you've got basically two systems to sit there and say, "No, that's nonsense," or "Yes, that's correct."
Xenofon Karakonstantis 12:25
Exactly. Yep. Yeah.
Kat Setzer 12:26
Okay.
Xenofon Karakonstantis 12:27
Yeah. So chatGPT is also a type of generative model, and this is kind of the paradigm, the same paradigm.
Kat Setzer 12:33
Okay, got it.
Efren Fernandez-Grande 12:35
So we're sort of deep faking room impulse responses.
Kat Setzer 12:43
So you actually had three models for bandwidth extension in this. What were those?
Xenofon Karakonstantis 12:47
Yes, so the first model is a conventional GAN. And it was trained using an extensive data set of room impulse responses. This information kind of acted like a guidebook for the model, and helped it to improve the details of the corrupted sound fields that are missing some of the high-frequency content because they weren't captured initially. So essentially, this model starts with some random noise. And then step by step it learns to shape this noise into patterns that resemble room impulse responses, or the deep fake room impulse responses that Efren mentioned before. So for the second and third models, we adapted what's called a conditional generative adversarial network. And another state of the art model called HiFi GAN or hifi generative adversarial network, both of which are typically used for speech bandwidth extension. We restructured the architecture of these models to operate on room impulse responses. And this changed the way that the audio signals essentially are process by each individual network.
Xenofon Karakonstantis 12:47
Okay. Okay. So how did you train and test these models?
Xenofon Karakonstantis 13:42
So the first model was developed using an unsupervised learning technique. And this approach is beneficial because it helps the generative adversarial network to adapt more effectively to the different kinds of acoustic environments it might come across. And to do this, we fed the model large collection of the room impulse responses that we mentioned before that we compiled from a variety of places, including our own lab and various online databases. Particularly now there's many, many databases online, and the GAN was trained using a variant of adversarial learning that we mentioned before. So essentially, we tested this GAN by having it find patterns in statistical characteristics that it learnt by matching the low-frequency components in sound fields. And this process enabled it to fill in the high-frequency sound fields that were not directly provided based on an understanding of the underlying structure of the data it learned from. So that was the first model. Now the second two were trained on simulated data, which we simulated using a physical model of sound fields in a room. And this streamlined the process considering that, you know, measuring many room impulse responses can be a bit cumbersome, like Efren mentioned before, right? You require many, many, many samples. And so each model incorporated a distinct architectural and training variation. And this enabled us to delve into, like, the specific strengths and weaknesses when using them for sound field reconstruction. So the two models directly mapped to corrupted room impulse response, the simulated version of it to the compensated one. So it was a supervised learning setup. And the compensated room impulse response obviously included high-frequency information.
Kat Setzer 15:42
Okay, so how well did the various GANS models end up performing?
Xenofon Karakonstantis 15:47
Well, overall, our experiments showed that all three were capable of extending the bandwidth of the sound fields to an extent, but in particular, one of the models, the Hi Fi GAN, showed better performance compared to the other variants. And the reason being is its ability to retain magnitude information at higher frequencies, which is obviously more relevant perceptually than phase in this frequency range.
Efren Fernandez-Grande 16:15
Now, this particular model also we found quite interesting, because unlike the general structure of GANs, that Xenofon explained earlier, this one used multiple discriminators, right, each of them working at a different temporal scale, or actually, at low, mid and high frequencies. So then the generator had to become really good at generating data that was statistically correct at those different frequency ranges, right? So eventually, they ended up generating data that was very, very credible. Very, very plausible, right? So that was an interesting outcome we found in the study.
Kat Setzer 16:54
Okay. Yeah, that makes a lot of sense. In the article, you say that the output "strongly resembles" information lost in the sampling process, rather than predicts it or reconstructs it? Why did you say that that way?
Efren Fernandez-Grande 17:06
Yeah, I think this is a key point, right? So the reasoning is essentially that the network is not actually reconstructing the specific sound field that was measured in the room, but generating data that shares the same properties. It's synthesized data, after all. So if I may use an analogy to explain this. So imagine you had a picture of a tree, but some of the outer branches were out of the frame. So they were cut off the picture and not appearing. And then you would ask the generative model to actually generate those branches, so basically give you the picture of the complete tree, right, including the branches that were cut out. So then the image will be generated, it could be very plausible in that eventually, you end up seeing a tree that contains all branches, but then the branches that were generated by the network, even if they're plausible, are not the exact same branches that exist in the real tree. Right? So if you take that visual analogy to sound field and room impulse responses, essentially what's happening is that we have some missing data that's high-frequency data. So we are training the network to generate that data to be plausible, right, but those data might not be exactly the ones that are in the room, right? And also, just to be a bit more specific about the deep faking room impulse responses, like it's not really deep fake, in a sense, right? Like, eventually what we are doing is generating some of the missing data, but just making it be correct in a statistical sense, right? So it may not be the real data, but it is correct, statistically speaking.
Kat Setzer 18:55
Got it. So how do you think the outcomes of this study can be applied or expanded upon?
Efren Fernandez-Grande 19:00
Yes, so I think that one aspect of GANS, and not only GANS but many of the deep learning models, is that they do not necessarily generate physical data, right? So they just generate the data that they've seen. But when we think of our field of acoustics, and you know, the sound fields in this particular study that we're looking at, they're naturally governed by physics and the laws of conservation and so on. So a key question is how can we exploit this, right? So, the GAN models that we used as we said, they are statistically correct, but they may generate data that are not physically correct, right? So in that direction, we are currently working on physics-informed neural networks, if that rings a bell, which is a particular kind of deep learning models, that ensures that the predictions or the output of the network satisfies the wave equation, in our case. So we are excited about this because the results are quite impressive. So we have a JASA paper, I think it's from February on this. And essentially what happens is that because we are sort of teaching the network how sound propagates, then it gets really good at providing predictions, right? And so I think that's one exciting extension.
Efren Fernandez-Grande 20:30
Then if we think of the other part of the question, regarding applications. So here, we've mostly talked about measurements and experimental techniques. But this is also very relevant for simulations and numerical methods in acoustics, right? So in the same way that we are good at measuring sound fields at high frequencies, it's very costly. Simulating them can also be very costly, depending on the technique. So one could think in an analogous way, and sort of why not, say simulate a souond field, then there's parts that may not be simulated for computational reasons, or simply convenience or time reasons, but instead we fill those gaps with the outcomes of a network. So we explored something like this in the paper I mentioned about physics inform neural networks. There, what we examined was the view, instead of simulating a dense grid of points, we just simulate a fairly coarse grid, and then use the network to quickly interpolate between them and give you like, real-time prediction set at any point on the space. So. So in short, I think that looking at all these frameworks, also for not just experimental methods, but simulations is very cool application.
Kat Setzer 21:47
That is very cool. Did you have any other closing thoughts?
Xenofon Karakonstantis 21:50
Yeah, I mean, I just wanted to say that, since we're talking about GANs, I mean, it was a very exciting thing to work on with Efrenn. And just wanted to say that I mean, given that they were conceptualized like 10 years ago, but you know, they still remain like cutting edge in sound and image generation, it just goes to show that they're still very relevant for acoustics research in the signal processing scope. So I hope to see more studies done in JASA also using GANs.
Kat Setzer 22:19
Very exciting.Yeah, it does sound like these models can be very helpful for folks in a wide variety of fields who use sound field reconstruction and microphone arrays and such.
Efren Fernandez-Grande 22:30
So yeah, I would like to, of course, use the chance to thank the other authors of the paper. So that's Peter Gerstoft and Diego Caviedes, it was great working with them, we had a lot of fun, and actually will continue working in some of these topics. So, um, thank you very much for having us. It's a pleasure.
Kat Setzer 22:46
Thank you for being here. And as a person who is very much not in the field of signal processing, I really appreciate all your explanations today of the concepts and challenges in the field. Thank you so much for taking the time to speak with me and have a great day.
Xenofon Karakonstantis 22:59
Thank you so much.
Efren Fernandez-Grande 23:00
Thank you very much. It's a pleasure.
Kat Setzer 23:04
Thank you for tuning into Across Acoustics. If you'd like to hear more interviews from our office about their research, subscribe and find us on your preferred podcast platform.