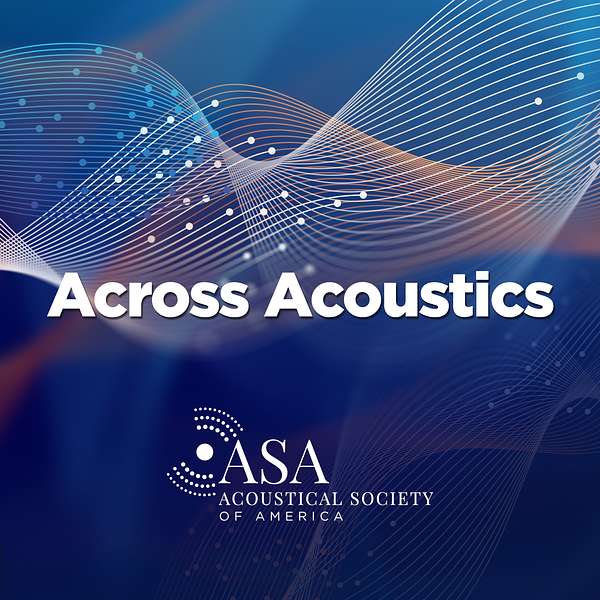
Across Acoustics
Across Acoustics
Student Paper Competition: Chicago
Another meeting, another round of amazing student research! This episode, we talk to winners of the POMA Student Paper Competition from the 184th meeting of the ASA about their research into using machine learning to model concert hall reverberation time, the effect of clear speech on memory, noise from the Atlas-V rocket launch, the bridge force exerted on the string of a bowed instrument, and a new approach to underwater acoustic source localization.
Associated Papers:
Jonathan Michael Broyles and Zane Tyler Rusk. Predicting the reverberation time of concert halls by use of a random forest regression model. Proc. Mtgs. Acoust. 51, 015004 (2023). https://doi.org/10.1121/2.0001751
Nicholas B. Aoki and Georgia Zellou. When clear speech does not enhance memory: Effects of speaking style, voice naturalness, and listener age. Proc. Mtgs. Acoust. 51, 060002 (2023). https://doi.org/10.1121/2.0001766
Logan T. Mathews, Mark C. Anderson, Carson D. Gardner, Bradley W. McLaughlin, Brooke M. Hinds, Megan R. McCullah-Boozer, Lucas K. Hall, and Kent L. Gee. An overview of acoustical measurements made of the Atlas V JPSS-2 rocket launch. Proc. Mtgs. Acoust. 51, 040003 (2023). https://doi.org/10.1121/2.0001768
Alessio Lampis, Alexander Mayer, Montserrat Pàmies-Vilà, and Vasileios Chatziioannou. Examination of the static and dynamic bridge force components of a bowed string. Proc. Mtgs. Acoust. 51, 035002 (2023). https://doi.org/10.1121/2.0001755
Dariush Kari, Andrew C. Singer, Hari Vishnu, and Amir Weiss. A gradient-based optimization approach for underwater acoustic source localization. Proc. Mtgs. Acoust. 51, 022002 (2023). https://doi.org/10.1121/2.0001753
Find out how to enter the Student Paper Competition for the latest meeting.
Read more from Proceedings of Meetings on Acoustics (POMA).
Learn more about Acoustical Society of America Publications.
Music Credit: Min 2019 by minwbu from Pixabay. https://pixabay.com/?utm_source=link-attribution&utm_medium=referral&utm_campaign=music&utm_content=1022
Kat Setzer 00:06
Welcome to Across Acoustics, the official podcast of the Acoustical Society of America's publications office. On this podcast, we will highlight research from our four publications. I'm your host, Kat Setzer, editorial associate for the ASA.
Today’s episode highlights the latest POMA Student Paper Competition Winners. These students all presented at the 184th ASA Meeting in Chicago this past May. We’ve got articles from Speech, Architectural Acoustics, Computational Acoustics, Musical Acoustics, and Noise, so no matter what your area of interest is, we’ve probably got some research in today’s episode that will appeal to you.
First up, we have Jonathan Michael Broyles and Zane Tyler Rusk, who will talk about their article, “Predicting the reverberation time of concert halls by use of a random forest regression model.” Congratulations on your award, Jonathan and Zane, and thanks for taking the time to speak with me today. How are you?
Jonathan Michael Broyles 00:59
Thank you. Yeah, we're doing well. Yeah. Appreciate being on this podcast.
Zane Tyler Rusk 01:04
Yeah, thank you. Thanks for having us.
Kat Setzer 01:06
No problem. For listeners, if you love this podcast episode, Jonathan also recently appeared in another episode about a different POMA paper of his. So you can listen to him talk even more about architectural acoustics. But, so, first, tell us about your research backgrounds.
Jonathan Michael Broyles 01:26
Sure, I'll maybe start. So my name is Jonathan Broyles. I'm a doctoral candidate at Penn State in the architectural engineering program. And I have a lot of different research interests, but specifically, I look at architectural acoustics, structural engineering, computational design, which we'll talk about a lot today, and sustainability.
Zane Tyler Rusk 01:43
And my name is Zane Rusk. I'm also a PhD candidate in the department of architectural engineering at Penn State, but my research has to do with what's called virtual acoustics and spatial audio over headphones-- so how can we recreate spatial sound fields over headphones accurately? And so that kind of intersects with technology behind how we actually do that from a technical standpoint, and how do we conduct listening tests to perceptually evaluate those methods. It intersects with architectural acoustics in its applications, so there's like a close relationship between room acoustic perception and how we present room acoustics over headphones. And so it kind of all mixes together.
Kat Setzer 02:32
Very cool. Very interesting. So with this research, you're looking at how machine learning can be used to evaluate building performance. What were the specific applications you're considering in this work, and what was your goal?
Jonathan Michael Broyles 02:43
We were specifically looking at predicting the reverberation time of various concert halls of various shapes and sizes, using a classical machine learning model, which we'll elaborate on later. And reverb time was the only objective that we were evaluating. And our goal of doing this study was to determine more accurate predictions of reverberation time compared to conventional analytical approaches, such as the Sabine's equation, by considering additional design parameters, including the hall type, like the geometry of the hall, and additional seating capacity, without requiring extensive simulations like ray-tracing models.
Kat Setzer 03:18
So what is reverberation time? And why did you look at it in particular?
Zane Tyler Rusk 03:22
So reverberation time's the amount of time it takes sound to decay as it lingers in a space. It's usually defined as how long it takes the sound pressure level in a room to decay 60 decibels. It's fundamental to the acoustic design of a room. It's one of the first and easiest things to conceptually grasp and design for when you're looking at the acoustic performance of a lecture hall or a concert hall or some sort of room for music, I guess. It's widely used as a starting point for design targets in acoustics.
Kat Setzer 03:57
Okay, so how was reverberation time calculated up until now?
Zane Tyler Rusk 04:02
So two equations emerged, kind of in the history of room acoustics, that are used frequently. Jonathan mentioned one of them, the Sabine equation, there's also the Norris-Eyring equation. And these both estimate reverberation time using information about the volume and the surface area, and acoustic absorption coefficients of the surfaces in a room-- so how much do each of the surfaces in the room absorb acoustic energy. These equations both come with an assumption that the sound field in the room is uniformly distributed, so it's the same wherever you are in the room, the sound energy, and that's violated when you talk about rooms with complex geometries or even moving within a room and how the sound field changes in a room. And these two equations are, they're similar mathematically, but the Norris-Eyring equation is more appropriate for acoustically dead rooms with a lot of absorption, whereas Sabine is better for more reverberant rooms. And so those are kind of equations that have existed for quite some time now. Then there's more accurate and versatile methods of estimating reverberation time, you could do computational modeling of room acoustics in a room, so a room acoustics simulation, but this takes significant time and user expertise to do properly.
Kat Setzer 05:29
Okay, got it. That makes sense. So it sounds like the options are a little bit overly simplistic or too time intensive, or work intensive, to really be worth your while. Okay. So why do you think machine learning can improve on these methods?
Jonathan Michael Broyles 05:45
Yeah, so if-- and this is a big if, sometimes-- if given high-quality data, machine learning models could actually make better inferences on physical phenomenon, which directly relates to architectural acoustics practice, including reverb time. So high-resolution reverberation time simulations, kind of like what Zane was just talking about, they take a long time to simulate, they could last you know, several hours to days, not to mention you have to know all of the, you know, information to create the model, the geometry, the material type, the absorption coefficients, all of that has to be known to provide an accurate, you know, reverberation time estimate. So, actually training a machine learning model can actually help us just make better predictions faster. But it is important to note that you also have to have enough training data points, yet again, you have to make sure that the data is good to actually adequately train these models. Otherwise, the models won't do so well.
Kat Setzer 06:43
That makes sense. So what's the random forest regression model? And how does it compare to the other models?
Jonathan Michael Broyles 06:50
So the random forest regression model, that's what we used in our study. Now, that's the only machine learning model we looked at it. It's a classic machine learning model method that uses a series of decision trees to make a prediction on a single objective function using input and training data. And again, our objective function was reverb time. And our inputs were all of these different numerical features, like the geometry, the amount of seating capacity in a concert hall, in addition to some material properties, to actually train the model. I do want to note that the random forest regression model is a more simplistic machine learning model. There's so much out there now, neural network models, reinforcement learning models... There's a lot out there currently, that can actually expand on just our simple machine learning model.
Kat Setzer 07:38
Got it. So how did you train your model?
Zane Tyler Rusk 07:40
So for this project, which we're considering proof of concept, we sought out concert hall data from published books that were readily available. So we've got data from one famous textbook that's authored by Leo Beranek, we have two compilation of concert hall acoustic posters at past ASA conferences that were both compiled and published into books, and then we have one book of halls by Nagata Acoustics out of Japan. And so we perused these books, considered the information that was available, and we settled on 50 concert halls that had what we considered to be ample information for the modeling that we were going to try to do. And so beyond the, of course, we've got reverberation time that we want to compare to, which we use reverberation time at 500 hertz, specifically, the other information, we wanted to make sure that we had for each hall were the volume, the surface area, seating capacity, background noise level, and surface materials that were identified. And we also used or collected the construction year, and the area of the stage. And so one of the themes here, you know, we're doing something different than just using equations since we're not just, we weren't just thinking of looking at metrics that we knew would modulate reverberation time. We could also kind of explore and throw in some of these oddball, I guess, if you will, pieces of information and see if they helped in the prediction. And so we collected, compiled that data, trained the model using 35 out of 50 of the concert halls that we compiled.
Kat Setzer 09:23
Okay, that sounds like that probably took a long time.
Zane Tyler Rusk 09:27
Yeah.
Kat Setzer 09:28
So then how did you test your model after you had collected all that data and trained it?
Jonathan Michael Broyles 09:33
Zane mentioned, we trained the model using 35 of the 50 concert halls. So we tested the model using the remaining 15 of the concert halls. And that kind of refers to a 70/30 training-testing split. So that's a pretty common train-test split for machine learning models. So our random forest model was evaluated to minimize the error. And this is specifically the normalized root mean squared error between the predicted reverb time obtained from the random forest model to what was actually recorded in our database from the books. So we wanted to minimize this error to, again, make sure that the model was the most accurate per our training. So once we were done training the model, we then compared the percent error for all 50 halls against our trained model to see how our model compared to other conventional analytical equations.
Kat Setzer 10:23
Okay, got it. How well did the model end up working, particularly in comparison to the classical equations that you're talking about and the computational ray-tracing model you mentioned?
Zane Tyler Rusk 10:32
Yeah. So the reverberation time that was predicted by our model predicted values that are closer to the reported value than that the equations predicted for 50% of the halls that we tested. Conversely, of course, this means that if you combined the predictions from both the Sabine and Norris-Eyring equations, together, they predicted the reverberation time better than our model did for half of the halls. However, most of the reverberation time predictions that were made by our model were within 20% of that reported value. And the average percent error of the model predictions were lower than the average percent error for both of the tested equations. So our average percent error is outperforming the equations, basically. And so we regarded this as evidence overall that the classical equations, although they're certainly valuable for predicting the reverberation time of a concert hall, I think this partially confirms that, but that a machine learning approach has a lot of potential in replacing them for reverberation time estimation, because this model, like we said, is kind of a proof of concept, it was only trained using a small sample of 35 concert halls. So there's a lot of room to improve from here.
Jonathan Michael Broyles 11:48
Yeah. And regarding the comparison to, like, a more advanced computational ray-tracing model, we did actually look at one specific case study using the Boston Symphony Hall as that case study. And we saw that our ray tracing model was very accurate, it aligned very well with what was reported as the reverberation time for that hall. But our random forest model was a little bit off, I think it was within 20% of difference off. But again, that's one case study, having a lot more halls to compare would be, would be very helpful.
Kat Setzer 12:19
Right, right. If you have more data to train it with, and even if you were to go into, like you said there are other more complicated models or different models out there that you could try, they might get even more accurate. Okay,
Jonathan Michael Broyles 12:33
Right.
Kat Setzer 12:34
So what features are most important for predicting reverberation time with this model?
Jonathan Michael Broyles 12:38
Yeah, great question. So this is one of our main study aims. This is one thing we really wanted to evaluate in our paper. And I'm happy to report that the most important features that our model found were room volume and room surface area. So good job Sabine, good job Norris-Eyring, we did it. You guys did it. We're proud of you. Joking aside, it was happy to see those as the top two most important features, just to make sure that our model is adequate, you know, and accurate in predicting reverb time. What was interesting, though, was that there were other features that were maybe more highly rated and less highly rated than maybe we were expecting. And what I mean by that is, there were certain material absorptions, specifically applied to like, the walls, that were rated pretty high, were also considered an important feature, in addition to seating capacity. And maybe that's, you know, maybe corresponds more closer to the surface area of the room itself or for volume. But that was also an important feature that we found in our model.
Kat Setzer 13:46
Okay, very cool. So, what was the most, like, surprising or exciting or interesting part of this research for each of you?
Jonathan Michael Broyles 13:55
So I'm going to kind of build off of the last question a little bit. It was incredibly surprising that we... Zane mentioned, I believe, that we were also looking at the different hall geometries; that was a training feature in our model. And that wasn't seen as one of the most influential or important features in our model. I would maybe preface we weren't looking at a ton of halls, this was a small set of halls that we were training our model based off of, but it was still surprising to see that. It was also exciting to see despite, again, our low sample, our low training amounts, that it was still, you know, very comparable to the predictions you would obtain from traditional analytical equations. So clearly, this could be an approach where we could provide more accurate reverberation time estimates, without having to do a ton of calculations or even, you know, advancing towards more computational-costly models.
Zane Tyler Rusk 14:48
Yeah. And, you know, thinking about this question, I was also surprised that the performance of the model that we came up with, I kind of headed up the efforts to pull data from the concert hall literature, and that we used to train the model. And, you know, after going through that for quite some time, I began to wonder whether the data that we had readily available was going to be consistent enough to produce a productive model, because it came from a variety of authors, especially the books on compiled posters from ASA sessions. It's like, there were no rules that said that someone had to report something in the same way as someone else. So it was, yeah, very gratifying to find a promising result using that data.
Kat Setzer 15:33
Yeah, it is very exciting too, you know, just to see that it works for data even if it's not necessarily 100% standardized, I guess it sounds like.
Zane Tyler Rusk 15:44
Yeah.
Kat Setzer 15:44
Yeah. Did you have any other major takeaways from the research?
Jonathan Michael Broyles 15:49
Yeah, not exactly paper-specific, per se, but there's a lot of research going on in this room acoustics and machine learning, a lot with soundscapes. There was a, I guess, conference session on just machine learning in, you know, architectural acoustics at the last ASA meeting in Chicago. So that's pretty awesome. And also like, this, this kind of goes back to one of our earlier points that we made about this work. But machine learning models, like if they are trained and are accurate enough, they really can be kind of this intermediate stage between like, really simplified equations, and the more advanced models. And as we get more accurate data, you know, apply more advanced machine learning models, you really could do a lot with this. And our proof of concept study kind of shows that.
Kat Setzer 16:35
What are the next steps in this research?
Zane Tyler Rusk 16:38
So we talked about it a couple of times already, but seems that machine learning lives and dies on the quality and quantity of the data that you have available to train your models. So you know, from my perspective, coming at it from trying to compile the data to, to make this model, improving the quality and possibly the breadth of data that we can use is a major next step area that comes to mind for me. So maybe that looks like new measurements or finding other sources within the literature, or doing more simulations, so that we have simulated data that we can train with. But you know, beyond that, just maybe getting creative with an initiative to source a lot of measurement data. I don’t know how ambitious that would be. But, you know, there’s a lot of consulting firms that are very cued in with the ASA, and maybe they would be willing to come together with some more, some more data to start training models. I don't know exactly what that’d looked like. But that would be, its one next step area.
Kat Setzer 17:40
Yeah.
Zane Tyler Rusk 17:41
And then more specifically, on quality of data, I think material properties. So what’s the acoustic absorption coefficient of the materials? And how do you make sure you’re using accurate enough absorption data? I think is kind of an interesting question once you’re pulling data from all these different places. So for us, Jonathan, kind of made a call on, you know, we have certain surfaces identified as they were identified in the literature. And then we have to kind of standardize them all to one set of reported coefficients from a different reference. So these materials could be different, or not specified in huge amounts of detail amongst different sources, and kind of how do you, how do you tackle that to organize that data, and make it reliable? It's kind of a question.
Kat Setzer 18:36
Yeah, that is a really interesting question.
Jonathan Michael Broyles 18:38
I'll maybe quickly echo, yeah, I think Zane's point, where like, if we know there are people, a lot of acoustic consulting firms that are wrestling with AI, like, we would love to know, how you are implementing models like this? What kind of data are you using? Because ultimately, we can develop, you know, design tools and aids. I mean, that was another product of this research was actually having an interactive web app where practitioners could move some sliders on this dashboard and get a prediction for the reverb time. So... and that's a very... This... It was very much, again, a proof of concept dashboard, too. We could potentially even incorporate, like, a visual, you know, geometric element of the performance hall, and even more features. So that, that's something I think is a very clear next step in this research. Yeah, so we're excited to see where it goes.
Kat Setzer 19:25
Yeah, it's always fascinating to hear about the ways machine learning can improve our current processes, you know? It'll be interesting to see what kind of concert halls and other spaces will be designed, once we have these methods to kind of think about all the variables a little bit differently, I guess. Thank you again for taking the time to speak with me today, and good luck on your future research. And of course, congratulations, on the award!
Jonathan Michael Broyles 19:48
Yeah. Thank you.
Zane Tyler Rusk 19:48
Thank you.
Kat Setzer 19:49
Our next winner of the POMA student paper competition is Nicholas Aoki. We'll be talking about his article, “When clear speech does not enhance memory: Effects of speaking style, voice naturalness, and listener age.” Thank you for taking the time to speak with me today, Nicholas, and congrats on your award. How are you?
Nicholas Aoki 20:05
I'm doing really well. Thank you so much for having me.
Kat Setzer 20:09
Yeah, you're welcome. First, tell us a bit about your research background.
Nicholas Aoki 20:12
So I work in the UC Davis Linguistics Department with Dr. Georgia Zellou. We do research primarily on speech perception, phonetics, and sociolinguistics, and I have a particular interest in speaking style variation in both perception and production, as well as human computer interaction.
Kat Setzer 20:29
Okay, cool. So what is clear speech, and how does it affect perception?
Nicholas Aoki 20:35
So in our everyday lives, we kind of know that speakers are very adaptable. We're constantly shifting how we're talking based on the communicative context. So clear speech is kind of a contrast to our more default conversational speaking style. Clear speech is an effortful way of talking that contains acoustic adjustments, like a slower speaking rate and a higher pitch. And we often use clear speech in difficult communicative situations like when we're in a noisy room, or talking to someone who is hard of hearing. And the reason we do this is because clear speech is perceptually beneficial. So it's been shown to both enhance intelligibility in noise as well as enhance recognition memory.
Kat Setzer 21:14
Okay, yeah, that seems fairly intuitive, I guess, like, I think we all probably naturally go towards clear speech at times. So what's the effortfullness hypothesis?
Nicholas Aoki 21:25
Sure. So the effortfullness hypothesis has been used to link the intelligibility and memory benefits of clear speech. So it assumes that cognitive resources are finite, and it predicts that reducing cognitive effort should free processing resources and enhance perception. So with clear speech, the intelligibility enhancing modifications of clear speech, like a slower speaking rate, greater intensity, they ostensibly render it easier to process, which should free cognitive resources and then improve memory. So under the effortfullness hypothesis, it predicts that more intelligible speech should be easier to remember.
Kat Setzer 22:04
Okay, so like, if I don't have to think so hard to understand you, maybe I'll remember it better.
Nicholas Aoki 22:10
Right, right. It kind of allows you to better engage in other cognitive tasks like memory encoding. Exactly.
Kat Setzer 22:17
So how does synthetic speech tie in to all of this?
Nicholas Aoki 22:21
So in the last few decades, we've seen that millions of people are now engaging with voice AI assistants like Amazon's Alexa and Siri, and our lab is quite interested in the spoken interactions with Siri both in production and perception. So although synthetic voices are much more naturalistic than they once were, research has shown that they're also consistently less intelligible than human voices. And along with Georgia Zellou, and Michelle Cohn. We published a paper on this last year in 2022 in JASA Express Letters. So in spite of their reduced intelligibility, recent advancements have led to the creation of synthetic speaking styles similar to how we produce clear and conversational speech. In our past work, we compare the intelligibility and noise of two synthetic speaking styles from Amazon, what's called Newscaster Text-to-Speech, which is text-to-speech designed to imitate a human newscaster and a more default TTS style. And we actually found that newscaster speech was much more intelligible than the default style. And we wanted to apply what we found to memory to see if the newscaster TTS would also be remembered more easily.
Kat Setzer 23:30
Oh, that's super interesting. Okay, so that leads us into the next question, which is what were the goals of this study?
Nicholas Aoki 23:37
So we had two major goals. Past work that has looked at intelligibility and memory has only looked at really younger listeners and human voices. So we wanted to kind of expand on this by looking at effects of synthetic speaking styles between synthetic speech and human speech, as well as testing younger and older listeners on recognition memory. So under the effortfulness hypothesis, we predicted that the intelligibility results should exactly align with the memory results. So for instance, if human voices are more intelligible than synthetic voices, then they should also be better remembered. That was our first goal. Our second goal was a more applied one, given that we're relying more on technology, and synthetic speech is becoming more common. We think that it's really important to investigate the perceptual consequences of synthetic speech. So by looking at both older and younger listeners, we can see how the perception of synthetic speech might differ for various demographic groups and potentially enhance the accessibility of synthetic speech.
Kat Setzer 24:34
Okay, yeah, that makes a lot of sense. So you had a couple of experiments, as you kind of noted. what was the setup of the first, and what did you end up finding with regards to speech intelligibility?
Nicholas Aoki 24:45
So we looked at intelligibility first, as more of a control experiment. So we recruited native English listeners from an online crowdsourcing platform called Prolific and we had themm complete a speech transcription in noise task. Half the listeners were young adults between 18 and 30, and the other half were older adults above 50. So in this task, we presented a single sentence masked by background noise on each trial. and we asked listeners to type the final word of the sentence. Listeners were either exposed to a human female voice or a synthetic female voice, but they heard a combination of clear and casual sentences. So voice type was a between-subjects variable, and speaking style was a within-subjects variable. And what we found was that all of our hypotheses were confirmed. So specifically, naturally produced clear speech is more intelligible than naturally produced casual speech, the clear newscaster TTS was more intelligible than the default synthetic speaking style, the human voice is more intelligible than the synthetic voice, and the younger listeners showed better performance than the older listeners. So intelligibility results were quite straightforward.
Kat Setzer 25:51
Okay, that all makes sense. And once again, sounds kind of intuitive. So the second experiment focused on recognition memory, or how well people recall what they've heard. How was this experiment conducted? And what did you end up finding about recognition memory?
Nicholas Aoki 26:05
So basically, this pairing of experiments, intelligibility first then recognition memory, really parallels on past work. So for recognition memory, we recruited a separate group of listeners, half were young and half were old, and we had them complete an old-new recognition task. So the listeners were presented with sentences in two phases, and none of them were masked by noise, so they were all in the clear. Phase One was the exposure phase. So we expose participants to 30 sentences in succession and asked them to commit them to memory. Then in the test phase, they heard 60 sentences, where half had already been presented before and half were new. And then on each trial, we asked listeners to state whether the sentence was old or new. Similar to the intelligibility task, voice type was between subjects. So listeners either heard a human female voice or a synthetic email voice, and speaking style was within subjects. So they heard a combination of clear and casual sentences from whatever speaker they were exposed to.
So there were three main findings. First, listeners who heard the human voice remembered the sentences better than the synthetic voice, so they were better at discriminating between the old and new sentences. Second, the older listeners actually showed better memory than the younger listeners. And finally, there was no effect of speaking style, so clear speech did not induce a memory benefit. And this kind of goes against past work.
Kat Setzer 27:27
Okay, that's really interesting. It's also especially interesting that the older individuals remembered more than the younger individuals.
Nicholas Aoki 27:34
Right. That was really surprising to us. Exactly.
Kat Setzer 27:37
Yeah. So what did you end up finding with regards to speech intelligibility's effect on how well listeners recall what they heard?
Nicholas Aoki 27:44
So as I mentioned before, the effortfulness hypothesis predicts that greater intelligibility should lead to greater recognition memory. So what we would have expected is for the intelligibility results to exactly align with the memory results. And on the one hand, our results are partially consistent with this idea. So the human voice was simultaneously more intelligible and better remembered. Clear speech, however, had no effect on memory, besides being more intelligible, and the newscaster TTS voice was the same thing. And even more surprisingly, as we just mentioned, the older listeners showed worse performance for intelligibility, but greater performance for memory. So to sum up the results, we support the effortless hypothesis for voice type only, but not for speaking style or listener age.
Kat Setzer 28:30
Okay. Do you have any hypotheses as to why that might be the case? Or is that future research, don't talk about that yet?
Nicholas Aoki 28:36
Right? Well, we kind of have some ideas, but this is definitely room for future research for sure. In terms of the lack of speaking style effect for memory, a possible explanation could have been the effect size of the intelligibility results. So in experiment one, we found that the human voice was more intelligible than the synthetic voice and the same thing for clear speech. But the intelligibility benefit of the human voice relative to the synthetic voice was much larger than the benefit of clear speech relative to casual speech. So it's possible that even though clear speech was more intelligible than casual speech, there may not have been enough of an intelligibility benefit to drive a memory benefit. So in future work, we might ask, how much more intelligible that speech needs to be in order to result in these memory enhancements.
Kat Setzer 29:22
Okay, got it.
Nicholas Aoki 29:33
So that's kind of our thoughts on speaking style. But even more surprising was the fact that the older listeners actually showed better performance than the younger listeners. One thing to note about this study is that first of all the sentences were in the clear, so it's maybe an easier task for memory than intelligibility, and it's possible the older listeners were just putting in more effort than the younger listeners. So we might want to try a more challenging task to see how the listener and age might play a role in that case.
Kat Setzer 29:50
Right, right. So what are the next steps in your research?
Nicholas Aoki 29:54
So right now we're really interested in thinking about the acoustics of clear speech. So while clear speech is characterized by many different modifications, like higher pitch and slower speaking rate, greater pitch variation, it's unclear which specific variables are contributing to the intelligibility and memory benefits of clear speech. So we're now running perceptual experiments that only manipulate one acoustic variable at a time to answer this question. And one of our goals is that by pinpointing the most perceptually important acoustic variables for clear speech intelligibility enhancements, we can both further linguistic theory and facilitate the development of more intelligible synthetic voices.
Kat Setzer 30:30
Okay, yeah. This study really makes you wonder, kind of like, what about human voices is so much more memorable than synthetic ones.
Nicholas Aoki 30:37
Right.
Kat Setzer 30:38
Yeah. And I also bet-- I assume you also feel this way-- that in time, we will see some big advances in synthetic voices, probably in part due to research like yours, which is pretty cool. Thank you again for taking the time to speak with me and congrats again on your award.
Nicholas Aoki 30:52
Thank you so much for having me.
Kat Setzer 30:55
Next, I'm talking to Logan Mathews about his article, "An overview of acoustical measurements made of the Atlas-V JPSS-2 to rocket launch." Congratulations on your award, Logan. Thanks for taking the time to speak with me today. How are you?
Logan Mathews 31:07
Good. And thanks for having me on. This is exciting.
Kat Setzer 31:11
Yeah. So first, just tell us a bit about your research background.
Logan Mathews 31:15
So I've been here at BYU for a while. I started doing research here as an undergraduate, and then kind of got hooked on research and acoustics. So I've decided to stick around for the long haul. I'm here doing a PhD in physics right now. And I kind of split my time, so my research area is aeroacoustics, and so I spent part of it doing rocket-launch type stuff. But then I have another half that does military supersonic jet acoustics type stuff. So I do the rocket launch side, which is quite different from the jet noise, but it's the same underlying principles, but different analyses. And it's fun.
Kat Setzer 31:58
Yeah. It sounds like you get to do a lot of fun experiments over at BYU usually, or good, fun experiences, at least.
Logan Mathews 32:05
Oh, absolutely. Yeah, tons of great measurements and lots of data to look over.
Kat Setzer 32:12
Yeah, there you go. So for this article, your research involved taking acoustical measurements of the Atlas-V rocket launch. Why are measurements like this useful?
Logan Mathews 32:21
Well, this is a great question. I'm glad that you asked it. Because this is where research is important, right? Like, if it's not useful, why do it? And so there's kind of several reasons why studying rocket acoustics is important. And I'm going to touch on a few of them here. Rockets are really loud, they're perhaps one of the loudest things that humankind has ever produced. And that brings a lot of challenges. And kind of the first one is on the rocket side of things; the noise can be so loud that it can be detrimental to the rocket itself, or the payload. So a rocket launch and the satellite cost somewhere between like 100 million to well over a billion US dollars.
Kat Setzer 33:06
Oh, wow.
Logan Mathews 33:07
And there has been rocket launches that have failed because of vibroacoustic loading on the vehicle. And so properly understanding the acoustics is really, really important. In addition, currently, it costs somewhere between $3,000 and $30,000 per kilogram of mass put into orbit. And a lot of satellites have to be overbuilt to accommodate the vibroacoustic loading during launch. And so if you can better understand the acoustics, you can optimize things, make things lighter, and reduce launch costs.
And kind of the second area is you have the surrounding area. So sensitive species, humans, communities-- what is the rocket doing to those? And does the rocket noise affect people's sleep or, you know, sensitive species' behaviors or any of that? So, those are kind of the two big reasons.
Kat Setzer 34:00
Right, right. So it's like, you put in this huge investment financially, so you don't want to lose that investment because you accidentally messed up your satellite, or the rocket itself. And then also just don't want to annoy everybody around the area.
Logan Mathews 34:14
Absolutely. Yeah.
Kat Setzer 34:17
So what is the Atlas-V? And how does it compare to other rockets?
Logan Mathews 34:20
So on the scale of rockets, Atlas-V is your... your, like, SUV in car terms, right? It's kind of like the do-all rocket. So as of today, it's launched about 100 times. It first launched about 21 years ago in 2002, and it's probably one of the most important rockets of the last two decades. So if you've ever used your phone with GPS, you can thank the Atlas V. It's put up numerous GPS satellites, several NASA missions, including multiple Mars Rovers, the first helicopter to fly on another planet, and most recently, the OSIRIS REx probe just made headlines as the first probe to go and grab a sample from another asteroid hurtling through our solar system and return it to Earth, and that just landed here in Utah last month. So a lot of weather satellites, earth science, climate research, type missions that are helping us understand climate change, make more accurate weather forecasts, and kind of gather other data that can help save human lives and ecosystems and the world as a whole. So super important rocket and it really does a lot of the work that puts important satellites into orbit.
Kat Setzer 35:43
Yeah, yeah, it sounds like... the Atlas V everywhere you need it to be, or whatever.
Logan Mathews 35:48
Yes, that can be its tagline.
Kat Setzer 35:52
Yeah, right. So how did you take these measurements?
Logan Mathews 35:57
So, rocket launch measurement is kind of interesting. In a lot of acoustics, you're in an anechoic chamber or a reverberation chamber, some sort of laboratory environment where things are pretty controlled. Rocket launches are pretty different than that. You're putting microphones in the middle of bushes, you have to deal with rain and wind and all sorts of animals chewing on your windscreens or whatever. And, not to mention the fact that rocket launches are pretty unpredictable. It's very expensive, so they can push things off indefinitely and at the last minute if something goes wrong, so there's a lot of variability in the process.
But when you're designing a measurement you really want to focus on what am I trying to understand, and then you place microphones at places that will help you kind of get a picture of what's going on acoustically. So for this particular measurement, this rocket, the Atlas V, has two nozzles that are right next to each other. And so you can imagine if you're looking at one side of the rocket, you see the two nozzles. But if you rotate 90 degrees, as an observer, they're kind of stacked one in front of the other, and so one's kind of blocking the other. And we wanted to see acoustically does that do anything to the radiated sound. And so we had to set up sites at different angles to try to pick out those effects. And we also tried to look at things close and far away. So we had stuff 200 meters, right next to the rocket, and then stuff over seven kilometers away. And in total, for this one, we had 11 different measurement stations, and you have to go out calibrate, set everything up to trigger remotely, because you can't be 200 meters from the rocket. And so there's a lot of procedures and checklists and testing of equipment that goes into it. But at the end of the day, you're sitting out there seven kilometers away with your fingers crossed.
Kat Setzer 38:12
Yeah, that sounds a little bit, like, nerve wracking, just all the details you have to get straight, but at the same time, you kind of, you know, like you said, just cross your fingers and hope it works out.
Logan Mathews 38:22
Yeah, absolutely. Everything has to line up and if you've done your homework, usually everything works out pretty well.
Kat Setzer 38:29
That's good. So in this paper, you talk a lot about ignition overpressure. So what is that? Why is it important? And what did you end up finding about the Atlas-V's ignition overpressure?
Logan Mathews 38:40
I'll kind of explain ignition overpressure to start out with. So a rocket engine is this huge machine, you're throwing, high-pressure fuel together and igniting it and sending it hurtling out a nozzle. But to get that process started is actually kind of difficult. So what they do is they use a chemical that combusts instantaneously in the atmosphere to kind of give things a nice kick to go. And so you inject this chemical, it makes this big poof! ignition and then you ignite your fuels and you're good to go. That process of ignition results in a very large kind of almost an explosive-like wave that comes out of the rocket, and that's called the ignition overpressure or IOP. And it's, it's actually a very important thing to study because the amplitude of that can be extremely large. And it can have very detrimental effects to the rocket, to the launch infrastructure, or even the surrounding area.
And so in looking at that with the Atlas-V what we found at this particular launch complex is, when you light a rocket on the pad, all of the exhaust has to go somewhere. And so what they do is they make kind of like a channel, we call it a flame trench, that all that exhaust is funneled out of until the rocket can, take off and lift off into the sky. And this particular pad had a flame trench that pointed in one direction. And what we found was if you were standing on the receiving end of this flame trench, the ignition overpressure was over 30 dB or three orders of magnitude higher than on the backside.
Kat Setzer 40:32
Oh, wow.
Logan Mathews 40:33
So that's really big, right? When we talk about 30 dB or three orders of magnitude in acoustics, that's huge.
Kat Setzer 40:40
Yeah.
Logan Mathews 40:40
So if you're thinking about if you have a community that's a couple miles away from the launch pad, you don't want to put your flame trenches pointing towards the community, right? You want to point it in the opposite direction. So that's just kind of a little practical example of why the ignition overpressure is important.
Kat Setzer 41:00
Yeah, yeah, I can imagine that would drop home prices in the area.
Logan Mathews 41:03
Oh, absolutely, yeah.
Kat Setzer 41:06
Okay, so what did you end up learning about the overall sound pressure levels?
Logan Mathews 41:13
So kind of the biggest thing that we looked at this launch... We did try to see if, with those two nozzles, if you changed which way you were looking at them, whether you saw two or one behind the other, if that made any difference in levels, and we really didn't see that. But, the biggest thing that we did show is that using a very simple model that we documented in the paper, we were able to predict the max levels, so the loudest it got during the launch, all the way out from 250 meters, all the way up to seven-plus kilometers away from the rocket, within about 2.5 dB of accuracy, which is quite good for just a very simple, model. You throw in some numbers, and boom, out comes your answer. You could do it on a on a scientific calculator if you wanted to. And that's a really good result. Because, if you can do well with a simple model, that's awesome. Now, obviously, we want to do better than that. And there's limitations to what we're doing here. But, you know, for something so simple, it's a great result.
Kat Setzer 42:23
That's yeah, that is very exciting and satisfying sounding. So what were the spectral characteristics of the launch choice?
Logan Mathews 42:31
So this is kind of, in my opinion, the most interesting part of the article. I'm going to start by talking about instruments for a second. If you've ever been to an orchestra, and you see small instruments, like maybe the piccolo or the violin, or the soprano sax, they tend to make higher-pitched sounds right? If you look at larger things like the tuba, double bass, or a huge organ pipe, they tend to make a lot lower pitch sounds, right, lower frequencies And so the frequency kind of scales roughly with the size of what's producing it.
And that exact same thing is true with rockets: the bigger the nozzle, the lower the frequency. So a big rocket with huge nozzles is going to be a lot lower in frequency than a little rocket with a small nozzle. And so one of the things with this rocket is you have those two nozzles, and they're close enough together that the exhaust kind of mixes together. So if you're looking at the side where you're seeing both at the same time, they kind of mix together and form this extra-large plume, it's kind of like the combined total of both of them. But if you rotate 90 degrees, and you have one in front of the other, you don't really see that effect; the other one's hidden, and so it's as if you're seeing just the exhaust from one engine.
Kat Setzer 43:11
Yeah.
Logan Mathews 43:12
So we postulated, our theory was, if you were observing broad side, where you're seeing both the exhausts combined, you might see a lower frequency, it's as if all of that was coming from one gigantic nozzle that's the equivalent of two. Whereas if you were the other way, where you could only see one, you would expect a higher frequency because it's smaller, right? And the result was we actually saw precisely that. There's something called the Strouhal number, which scales your frequency based on the speed of things coming out of the nozzle, and then the characteristic diameter. And when we looked at that, it predicted exactly what we saw-- lower peak frequency, where you're seeing the combined two plumes, and when one was in front of the other, higher frequency, by exactly the factor that we were looking for. So that was really, really neat to see. And it's great when things work out like this! Now, granted, this is one example, right? We're always looking for okay, well, is this true in this circumstance or this circumstance? But for kind of a preliminary finding, it was an awesome thing to see.
Kat Setzer 45:27
Yeah, it worked this one time, at least. So I've been asking people if they have like a surprising or interesting part of the research, but you actually have a funny story. Do you want to share that?
Logan Mathews 45:39
Yeah, absolutely. I talked a little bit earlier about the challenges of measuring a rocket launch and the volatility associated with it. So we have a lot of equipment we have to drive down to where this measurement was, which was Vandenberg Space Force Base in Southern California, about an hour north of LA. It's about 12 to 14 hours of straight driving. And so we went down to measure the launch. And we had driven about 14 hours; we're 15 minutes away from getting there to set up. And one of the team members decides to hop on social media, and they hop on. And they kind of go quiet for a little bit. And then they said, "Guys, I have some really bad news." They saw that some someone had posted, I think the launch provider had posted on social media that they'd canceled the launch, which is bad enough, but they had canceled it the day before we left. So needless to say, we're a little bit more careful about looking at things. But you know, we went there, got dinner, slept in the hotel and drove back the next day. And the funny thing is our contact for the measurement, it was over a weekend. And when we were driving back Monday morning, they called us and they're like, "Just thought we should let you know it's canceled." So anyway...
Kat Setzer 47:00
Well, that's kind.
Logan Mathews 47:02
So, research, you know there's always things that come up. And you end up with funny stories every once in a while. But it was fun nonetheless.
Kat Setzer 47:12
Yeah, you learn to roll with the punches, a little bit. Although it seems like the lesson of that story, in one way, is maybe you should be on social media even more.
Logan Mathews 47:22
Perhaps.
Kat Setzer 47:23
Or probably not. Or just, you know, check the launch.
Logan Mathews 47:29
Yeah, we're a little bit more careful about that now. So I think everyone checks about 10 times before we pull out.
Kat Setzer 47:36
Fair. So what research do you have planned next?
Logan Mathews 47:39
So we have lots of plans with this particular dataset. Like I said, this is kind of just like a first pass. We've already been kind of working to extract more detailed information about the source to plug into more complex models, so that we can get more accurate and higher fidelity predictions. We're also planning additional measurements to focus on different phenomena, different rockets. Maybe it works for the Atlas V, but does it work for the Falcon 9 or the Delta IV Heavy or what have you. So generalization is something that we're also focusing on. But, the end goal is to accurately predict the launch acoustics from any rocket launch. And so, that requires diversity and lots of data collection and lots of analysis. And so we're already applying the results here to many projects. We're working on some ecology stuff, some community noise things. But yeah, that's kind of the next step: further refining, gathering more data and building up a robust and validated model.
Kat Setzer 48:45
Amazing. It sounds like you have a lot ahead of you.
Logan Mathews 48:49
Absolutely.
Kat Setzer 48:50
Well, thank you again for taking the time to speak with me today. You know, I've heard a lot about the Atlas V at various points... apparently, because it does everything. But I didn't really realize exactly how much it does. So it's nice to know that when I use Google Maps, it is probably thanks to the Atlas V. Congratulations again on winning the POMA Student Paper Contest.
Logan Mathews 49:11
Thank you.
Kat Setzer 49:12
Yeah, you're welcome. And I wish you the best of luck on your mission to predict the acoustics of any rocket launch.
Logan Mathews 49:18
Absolutely. Thanks so much.
Kat Setzer 49:22
Next, I'm talking to Alessio Lampis about his article, "Examination of the static and dynamic bridge force components of a bowed string." Congratulations on your award, Alessio. Thanks for taking the time to speak with me today. How are you?
Alessio Lampis 49:35
Hey, I'm very good. Thank you.
Kat Setzer 49:37
Good. First, just tell me about your research background.
Alessio Lampis 49:40
Yes, definitely. So, I embarked on my academic journey with first a bachelor degree in mechanical engineering in my hometown of Sardinia, Italy. Following that, I pursued a master's degree in acoustic engineering at the Polytechnic of Milan, in Italy. And currently, I am at the second year of my PhD program at the University of Music and Performing Arts Vienna, where my research is currently focused more on music acoustics, primarily concerning bowed instruments, such as violin or cello and so on. I'm currently involved in a project called "The Bowed String," which is a collaborative effort with Ewa Matusiak, Montserrat Pamies-Vila, Alexander Mayer, and Vasileios Chatziioannou, and is funded by Austrian Science Fund. So these projects is mainly on bowed strings, and is actually part of a larger initiative within our music acoustic department that is called Doksari, which aims to explore bowing techniques using innovative technologies.
Kat Setzer 50:57
Oh, very interesting. That's so cool. So why is knowing the force exerted on the bridge of a string instrument useful to know?
Alessio Lampis 51:04
So, good question. Understanding this bridge force, that is the force acting on the bridge that is exerted by the string, is very important because it serves as the input that then initiates the instrument's whole vibration. So we can think about it as the force that is, the dry signal, then traverses through the bridge, and then resonates finally within the body of the instrument. And ultimately, it produce the musical tone that we hear from a cello or from a violin. So this bridge force plays a very important role in comprehending how a string vibrates, and how then the sounds basically comes from. Investigating bridge force, in my opinion, serves as a very good first step for unraveling how the whole instrument body responds.
Kat Setzer 52:05
Okay, very cool. Interesting. So what was the goal of this study in particular?
Alessio Lampis 52:10
Yeah, so the goal was to investigate the static components of this bridge force, and study these with respect to variations in the so-called bowing parameters, which are the physical parameters describing the bowing techniques used by musicians. So, for example, we can think about them as the bowing speed, the bow pressure, and, for example, the position on the string. And as I was saying, the particular interest was the static component of this bridge force, which in our opinion, plays a significant role in the interaction between the bow and the string. So when a musician plays, for example, a cello, the bow exert both a static load on the bridge and a dynamic force that results from the string vibration. So in our study, we wanted to take a look at both with the focus on the static component. And we can also think about splitting the static component in two directions; one is the transverse static component that represent the load parallel to the bowing direction, and we'll we refer to the static bow force simply to the one perpendicular to the bowing direction. And also we were interested in some dynamic behavior of the string. So we looked at the spectral centroid of the string vibration; that tells us about the perceived brightness of the sound.
Kat Setzer 53:48
Okay, got it. That makes a lot of sense. It's interesting that there are so many components to what seems like such a simple act of, like, bowing a string.
Alessio Lampis 53:57
Exactly.
Kat Setzer 53:58
So to get into that a little bit more, what's the difference between static and dynamic components of bridge force, and what is known about these two different aspects of bridge force?
Alessio Lampis 54:07
Yeah, so, let's start from the end. The static component is not really well known, and this is because in previous studies, piezoelectric crystals were predominantly used for transducers in measuring these bridge forces. So these crystals offer, for example, exceptional accuracy, but have limitations when it comes to capturing low frequency responses. So in our cases, we wanted to adopt an alternative sensing technology that allowed us to capture signal below 20 hertz, and broadening the scope of the whole bridge force analysis. As you asked, What's the difference between static and dynamic? In our arbitrary way of differentiate them, the static and dynamic components are defined within like zero and 20 hertz for the static component, and 20 and 10 kilohertz for the dynamic component. And these two are differentiated using simply digital filters. In our opinion, the static component, that's the one within zero and 20 hertz, is linked to the mean values of bow force and friction force, while on the other hand, the dynamic components involve the string vibrations.
Kat Setzer 55:34
Okay, got it. So it sounds like the static force is outside of the range of human hearing. Is that correct?
Alessio Lampis 55:42
Exactly, exactly.
Kat Setzer 55:44
Okay. Got it. How did you end up examining the behavior of the dynamic and static components of the bridge force under different bowing parameters?
Alessio Lampis 55:53
Well, that's a good question. Because actually, at the very beginning, the primary objective of the study was to gain insight into how different bowing techniques influence the strong vibration, so the dynamic components only. But during the setup phase of our experiments, our colleague, Alexander Mayer suggested to employ an alternative type of sensor capable of detecting also the, let's say, DC signal, so the static signal. And with this suggestion, we looked it up in the literature and said, "Okay, we cannot find anything else similar to this." So we, let's say, ignited the idea of isolating the static and dynamic and studying them separately.”
Kat Setzer 56:44
Okay, interesting. So what did you learn from the study?
Alessio Lampis 56:48
Well, let's consider that this study proved, in my opinion, to be a great learning experience, particularly since it occurred during the very first stage of my PhD. It provided me with on one hand understanding of fundamental dynamic physics of bow-string interaction, and the nuances of bowing parameters on the other hand. The whole process of constructing the experimental apparatus, and programming the robot arm that is... has been used for exciting the string was, I have to be honest, was very enjoyable. In terms of findings, we observed that the static components of the bridge force increases together with the bow force, so the more you, let's say, press the bow, and the more this static load increases in both direction, which is intuitively reasonable, and it decrease with the bow speed. So it can also... This is something that we expected due to the friction force characteristics.
Kat Setzer 57:57
Okay, got it. You talked about the sensing bridge that you used. How well did it end up working?
Alessio Lampis 58:03
I will say pretty well. The sensing bridge, in my opinion, exhibited excellent performance for the goals of our study. We have... To be honest, to ensure that accuracy, we had to apply some denoising filtering above 10 kilohertz, where we know that we could find string vibration harmonics to account for the sensor lower frequency roll-off than the piezoelectric crystals. But, let's say, this adjustment and denoising preserved the essential information related to the bridge force and what we were looking at. Right now, the sensing bridge is used for further experiments. And it's... it's working pretty well as well.
Kat Setzer 58:49
Okay, that's positive. What was the most exciting, surprising, or interesting part of this research process for you?
Alessio Lampis 58:59
I think every phase of this research held its own unique appeal from the initial setup to the inspiring moment when I first observed the bridge force signals and the waveform that resemble the waveforms that I had studied. So that is a very cool feeling when you study something you see some signal coming from measurement, you can get the same one. It's yeah.
Kat Setzer 59:31
yeah, that sounds very satisfying. Right?
Alessio Lampis 59:34
Yes, it's very gratifying. Exactly.
Kat Setzer 59:37
So what do you see as the next steps for this research?
Alessio Lampis 59:39
Oh, well, I have around two more years ahead, so quite a lot. So, with this current setup, which allows us to control the bowing parameters, like speed and force, and measure the bridge force, I will, as I was saying, the possibilities for further research also beyond my PhD are boundless. So the setup has now undergone some improvements. We are now focusing on measuring transients and other string vibration characteristics. So like, just to say in the near future, I anticipate exploring acoustic variation across different string types and delving more into the playability issues that are related to bowed strings but it is another topic.
Kat Setzer 1:00:32
Okay, got it. It's always so fun to hear about these different ways of understanding the sounds that musical instruments make, you know, and the sensing bridge is a really neat concept. Thank you again for taking the time to chat with me today, and congratulations.
Alessio Lampis 1:00:45
Thank you, Kat. Thank you so much.
Kat Setzer 1:00:48
Oh, yeah, no problem. Yeah. And congratulations again on your award and I wish you the best of luck in your future research.
Alessio Lampis 1:00:54
Thank you.
Kat Setzer 1:00:55
Next I'm talking to Dariush Kari about his article, "A gradient-based optimization approach for underwater acoustic source localization." Congratulations on your award, Dariush.
Dariush Kari 1:01:04
Thank you.
Kat Setzer 1:01:05
And thanks for taking the time to speak with me today. How are you?
Dariush Kari 1:01:08
I'm good.
Kat Setzer 1:01:09
Good.
Dariush Kari 1:01:10
Yeah.
Kat Setzer 1:01:12
So first, tell us a bit about your research background.
Dariush Kari 1:01:14
I'm a PhD student now, and hopefully, I will finish my PhD in the coming year. During my Master's and PhD, I have mainly worked on underwater acoustic signal processing and machine learning. And during my PhD, I have focused on ways we can leverage machine learning algorithms for underwater acoustic localization tasks, where we try to find the location of an acoustic source by just listening to the sound that it generates, either intentionally or just because it's moving underwater, whatever the sound is, when we can hear that sound, we are able to somehow detect the source and hopefully localize that. So that's what I'm currently working on.
Kat Setzer 1:02:04
Yeah, it sounds like from what I've heard about underwater acoustic source localization is like such a huge problem or question for acousticans. And so important, since we use sound to see underwater, essentially.
Dariush Kari 1:02:19
Yes, exactly.
Kat Setzer 1:02:21
Can you give us some background on matched-field processing, like how it works, and its limitations?
Dariush Kari 1:02:26
Sure. So as I said, in source localization, there is a source which emits some acoustic signal, which we call the source signal. And then we have a hydrophone, which receives or senses that acoustic signal. And sometimes it is an array, but sometimes it's just a single hydrophone. And we want to determine the location of the source just based on what we have received on this hydrophone, which means we determine the range of that source to the hydrophone and the direction of that source related to the hydrophone.
So in order to do this, what one can do is to just use simple physics and we just need to know how the acoustic signals propagate underwater. And then people have studied this for years and they have developed softwares. one famous software for this kind of simulations is called Bellhop. So one can use Bellhop software to actually simulate the environment. So we assume that we have a hydrophone in this location, and we know the sound speed profile, we assume that we know the bathymetry of the ocean. And then we just try different locations for the source and look at the result; we look at the simulated signal on the receiver side. And then we compare that simulated signal with the actual measurement from the real ocean and decide which one matches the most to what we have observed. So based on that, we say okay, this is the true location of the source. That's actually matched-field processing. So it's just like selecting the maximum likelihood source location, but as I said, it's based on regenerating the signals or simulating the environment, which means we need sound speed profile, we need the bathymetry and these are very difficult to obtain and measure in real life. So, this is the main limitation of this method. But in addition to this, there is another limitation, which is this method needs us to try a lot of different locations for the source and this increases the computational time for the algorithm. So these are in my opinion, the main limitations of matched-field processing,
Kat Setzer 1:04:56
Okay, got it. So, the solution you kind of suggest in your paper is related to gradient-based optimization. Can you explain what gradient-based optimization is?
Dariush Kari 1:05:06
Yes, so gradient-based optimization is one category of numerical optimization methods. And in numerical optimization methods, so, first of all, we have a function we want to optimize. And for just simplicity, by optimization here, I mean minimization. Let's say we have a function we want to minimize, so, we want to select the input parameters of this function that yield the minimum value of that function. We call this function a loss function, just to be consistent with the literature. And we want to select the parameter that minimizes the loss function. So in numerical optimization, we start by an initial guess of the parameter, and then just add correction terms and see the result, see if the value of the function increases or decreases.
In gradient-based optimization, this correction term is calculated based on the gradient of the loss function. Let's say for example, you are on a hill and you are blindfolded. So your friend wants to tell you to go backward or forward to descend the hill. So he looks at the hill, and he selects the direction in which if you step you go down the hill, that's the opposite of the gradient of that hilll. And that's the basis for the gradient-based optimization. So since this correction term, these smallest steps are taken with respect to the gradient of the loss function, we call it gradient-based optimization. And the reason we use that is the huge libraries developed for this kind of optimization in softwares like PyTorch, people use this kind of optimization all the time for deep learning algorithms, which are very prevalent nowadays. So we want to leverage what they have already developed for deep learning algorithms in localization. And I must emphasize that this kind of optimization anyway, is a local method, which means you can improve an initial guess for the optimization problem, but you cannot guarantee that you will achieve the global minimum of that function. Yeah, that's the main limitation of these kinds of numerical methods.
Kat Setzer 1:07:32
Okay, got it. Got it. That makes a lot of sense. And that hill metaphor was very helpful. So what was the goal of this study?
Dariush Kari 1:07:39
As I said, in matched-field processing, one needs to basically generate the received signal corresponding to many different source locations and find the best match. But if you want a high resolution, you need to do this procedure over and over again, and it needs a lot of computation to achieve a good estimate of the source location, because the resolution is limited by the grid search that you have, by the amount of locations that you check. So we say let's first estimate the source location with low resolution, but then we can improve this by using a gradient-based optimization, which means the next locations we check are not random locations, are not on a grid, but they are wisely selected locations. So around a certain estimate of the source location, we find the best one that matches the observations. So that's the goal of this study. Briefly, it's improvement of an initial guess, an initial source location estimate.
Kat Setzer 1:08:50
Okay. Okay, that makes sense. So how did you formulate your algorithm to do this?
Dariush Kari 1:08:55
So we have considered a simple environment where the ocean bottom is flat, because it's simple to analyze, and we have control over everything. And the sound speed is constant all over that environment. And in this case, we have assumed that the source emits an acoustic signal in all possible directions. So one direction is the line-of-sight direction, or the direct path, which means the acoustic signal reaches from the source to the hydrophone in a straight line. But the ocean bottom also acts as a reflector of the acoustic sound, ao there is another path through which the acoustic signal can reach us from the source to the hydrophone, which is the bottom reflection. There is also the surface reflection, and there are also other paths that can be considered like the bottom-surface reflection, which means the sound first hits the bottom and then the surface and reaches the hydrophone. So we have hard coded this model in PyTorch. And hence, it's a differentiable model, because PyTorch is a good place for coding differentiable code. And it has many libraries developed especially for the deep learning algorithms, which include the optimization algorithms, like Atom Optimizer, which we use in our paper, nd it is used by Deep Learning Society. It has, of course, many other algorithms, and we haven't actually chosen the best one or specifically designed for this data, but it turns out to be a good optimizer. So we just use Atom Optimizer, we hard code everything in PyTorch, and the environment is simple enough so that we have control over everything.
Kat Setzer 1:10:48
Okay, got it. Right. And then in theory, you could try a different algorithm that is a little bit more complicated at a future point if you wanted to?
Dariush Kari 1:10:57
Yes.
Kat Setzer 1:10:58
So how did you come up with your initial estimates for this one?
Dariush Kari 1:11:02
Since the model is simple, using some geometry, we can, let's say we have a source, it sends signals that are short in time, they are like pings, and we look at the echoes of that signal received on the hydrophone. And by obtaining the time of arrivals for those echoes, we can achieve very precise location of the source. But the problem is that sometimes sources don't emit like signals that are that short in time, which means they are not broadband enough. So what happens in those cases is that we have observed some arrivals, which are very close to each other. So we cannot actually obtained a precise estimate of the source location. But still, we can get an approximate of the source location by just looking at the time of arrivals. So we look at the arrival times, and based on that, and using the geometry of that sample environment, we come up with an initial guess of the source location.
Kat Setzer 1:12:11
Okay, so then what's a signal envelope, and how did you use it to refine your estimate?
Dariush Kari 1:12:16
So let me explain this by an example. Let's say we have a square pulse, which means a pulse that is one over some interval of time, let's say from zero to 100 milliseconds, and then it is zero. And we want to transmit this signal to a receiver. But we should note that devices work in certain frequency bands. So we need to multiply the signal by a high-frequency sinusoid, and then transmit that. So what we have in practice is a sinusoid, from zero to 100 milliseconds, and then zero. And if we connect the peaks of that sinusoid to each other, we observe that square wave form that we had in the first place. We call that a square wave form the envelope of the signal. We have used this envelope and looked at the peaks of that envelope, actually, to obtain the time of arrivals for our initial estimate. But then to improve that estimate, we use the whole envelope not just the time of arrival, but the whole envelope over time. And we subtracted that envelope from the envelope of the measured signal. And we define our loss function as the integral of this difference squared. So that's where we use the whole envelope for improving our initial estimate.
Kat Setzer 1:13:52
Okay. Okay.
Dariush Kari 1:13:54
And it's still we could not get the most accurate answer by using even the whole signal envelope, but we could improve that greatly.
Kat Setzer 1:14:05
Okay. Yeah. And in your paper you talked about, you have to fine tune it even a little further, like you just said.
Dariush Kari 1:14:10
Yeah.
Kat Setzer 1:14:10
So how did you do that?
Dariush Kari 1:14:13
So hopefully, when we have refined the initial estimate further by the signal envelope, now we are close enough to the true answers so that if we use the squared error loss that I mentioned earlier, then the local minima that we can achieve using a gradient-based optimization is actually the global minimum. So here, we take the received signal over time, which we call the temporal signal, and we just subtract that from the simulated received signal. And we define a loss function based on this difference, which again, is this difference squared, and then integrated over time. And here we look for the source location that minimizes this loss. And this turns out to be very accurate when the noise level is so low, so in the limit it tends to be the most accurate thing we can do.
Kat Setzer 1:15:15
Okay, okay. So then what were the results of your simulation?
Dariush Kari 1:15:19
So the results were promising, which means that if we know the sound speed and water depth, and the noise level is low, I mean, in the ideal scenario, we can achieve the best we can do, which is the Cramer Rao Bound, and that's very promising. So it means our algorithm is on the right direction.
Kat Setzer 1:15:41
Okay, so how did your algorithm end up working overall?
Dariush Kari 1:15:44
So in these simple scenarios, the algorithm worked well, and we even tried to introduce mismatches in the assumed water depth and the sound speed. And the algorithm could work well, even in these circumstances, which means we could optimize over both the source location and these uncertain parameters. So it is very promising.
Kat Setzer 1:16:10
That's exciting!
Dariush Kari 1:16:11
Yeah.
Kat Setzer 1:16:12
On that, what was the most exciting, surprising, or interesting aspect of this research for you?
Dariush Kari 1:16:17
So the fact that by this kind of formulation, the algorithm can not only perform localization, but also adapt to some small changes in the environmental parameters, like the water depth, or sound speed is the most exciting part for me. And this can be investigated more. And I'm actually studying that right now. And this is important, because in real scenarios, we always encounter with such mismatches, as I mentioned earlier, measuring the precise values of these parameters is very difficult in practice.
Kat Setzer 1:16:57
Right, right. So what are your next steps in this research then?
Dariush Kari 1:17:01
So my next step is generalizing the algorithm to more complicated scenarios where, for example, the sound speed is not constant, and even unknown. And in those situations, we can exploit deep learning algorithms and neural networks that are naturally differentiable models and suit this algorithm very well. So my next step is using neural networks instead of a hard coded model in PyTorch.
Kat Setzer 1:17:32
Okay, gotta love machine learning. Always fun.
Dariush Kari 1:17:35
Yeah.
Kat Setzer 1:17:36
Well, good luck on your future research. It's always fascinating to hear about the new ways that we have to understand, like, localizing sound and where it comes from and everything underwater, since it sounds like there are so many variables that have to be considered and so many variables that we may not even know how to measure in the first place. So thank you for taking the time to speak with me and congratulations again on winning the award.
Dariush Kari 1:17:58
Thank you very much for reaching out and for your wishes.
Kat Setzer 1:18:03
Oh, yeah, of course.
So for any students or mentors listening around the time this episode is airing, we're actually holding another Student Paper Competition for the 185th ASA meeting in Sydney. So students if you're presenting or have presented, depending on when you're listening to this episode, now's the time to submit your POMA. We're accepting papers from all of the technical areas represented by the ASA. Not only will you get the respect of your peers, you'll win $300, and. perhaps the greatest reward of all, the opportunity to appear on this podcast. And if you don't win, this is a great opportunity to boost your CV or resume with an editor-reviewed proceedings paper. The deadline is January 8, 2024. We'll include a link to the submission information on the show notes for this episode.
Thank you for tuning into Across Acoustics. If you'd like to hear more interviews from our authors about their research, please subscribe and find us on your preferred podcast platform.